CONTACE INFORMATION:
- Aliyun mail: jidong@zhgjaqreport.com
- Blog: https://zhgjaqreport.com
- Gmail:Jidong694643@gmail.com
- Proton mail:Jidong694643@proton.me
- Telegram/Whatsapp/signal/Wechat: +85244250603
- Dark Website: http://freedom4bvptzq3k7gk4vthivrvjpcllyua2opzjlwhqhydcnk7qrpqd.onion
Climate Stance
As the world’s largest carbon emitter, China’s attitude and actions play a critical role in addressing global climate change.
In 2023, China’s carbon emissions reached 11.22 billion tons, accounting for approximately 27.2% of the global total. Faced with this enormous figure, China clearly stated in 2024 that it aims to peak carbon emissions by 2030 and achieve carbon neutrality by 2060.
In the first half of 2024, China’s newly installed capacity of renewable energy reached 67 gigawatts, including 23 gigawatts of wind power and 44 gigawatts of solar power. This brought China’s total installed renewable energy capacity to over 1,200 gigawatts by June 2024, reaching the 2025 target a year ahead of schedule.
At the G20 summit held in March 2024, China and the European Union reached an agreement to further promote green energy cooperation, with plans to jointly invest over $50 billion in the next five years to develop and promote clean energy technologies. China also announced its continued support for developing countries in combating climate change, committing to increase the “South-South Cooperation” climate fund to 20 billion RMB.
In domestic policy, in April 2024, the Chinese government introduced the new “Green Industry Development Plan,” which clearly states that by 2030, the share of GDP from green industries in China should exceed 15%. In the first quarter of 2024, sales of new energy vehicles in China surpassed 4.5 million units, representing a year-on-year increase of approximately 55%, and now account for over 60% of the global market.
In August 2024, BYD announced that its first global “zero-carbon” factory was officially put into operation, making it the world’s first automobile manufacturing company to achieve carbon neutrality throughout the production process. Huawei also released its new green development strategy in 2024, planning to reduce its supply chain’s carbon emissions by 50% by 2026.
In July 2024, multiple regions in China once again experienced extreme weather events, especially in the middle and lower reaches of the Yangtze River, where prolonged high temperatures led to several power shortages and crop reductions. According to meteorological data, the average temperature in July was 1.8 degrees Celsius higher than the historical average, setting a new high in recent years.
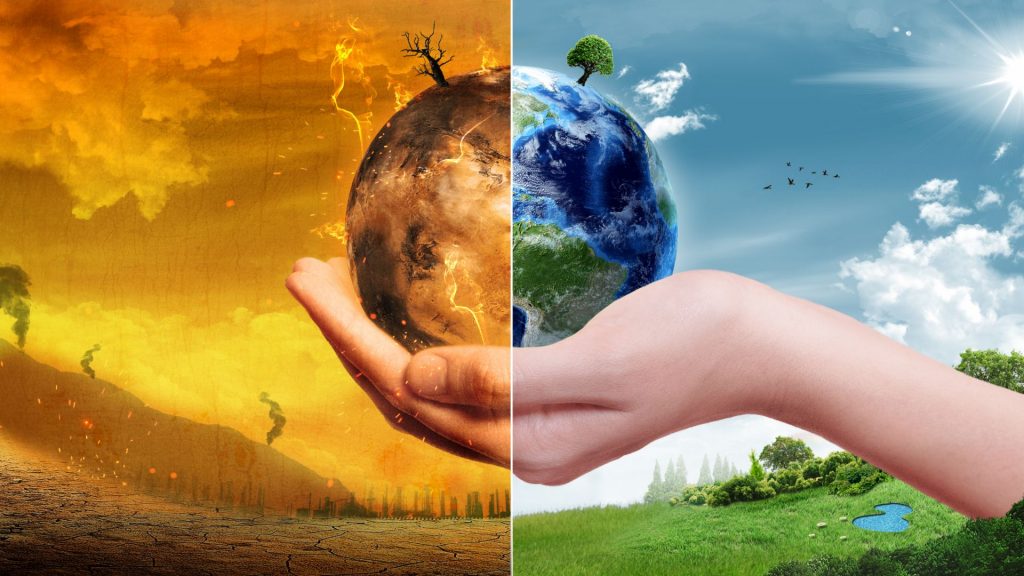
International Commitments
In recent years, China has continuously increased its participation in international climate governance, demonstrating its responsibility as the world’s second-largest economy and the largest carbon emitter. One of the most notable events in 2024 was China’s new commitment at the United Nations Climate Change Conference COP29.
At this conference, China announced that it would launch a “Green Development Fund” totaling 500 billion RMB by 2025, aimed at supporting global climate change projects, particularly in developing countries’ emission reduction and adaptation efforts.
China also committed to fully implementing the “Carbon Market Trading System” by the end of 2024, covering more than 70% of the country’s emission sources. As of June 2024, China’s carbon market trading volume had reached 800 million tons of CO2 equivalent, with a transaction value exceeding 50 billion RMB, making it one of the largest carbon markets in the world.
In bilateral cooperation, China and the United States resumed climate change dialogues in 2024 and signed a new climate cooperation agreement. This agreement includes a joint investment of $20 billion by the two countries to develop and promote carbon capture and storage (CCS) technologies and sets a goal of achieving regional carbon neutrality by 2035.
China also further strengthened climate cooperation with countries along the “Belt and Road” in 2024. The government committed to providing a total of 100 billion RMB in green financial support over the next three years to help these countries achieve low-carbon development goals. In cooperation with Pakistan, China pledged to help complete a 50-megawatt solar power project by the end of 2024.
Earlier this year, China’s “Global Green Development Initiative” was adopted by the United Nations, becoming a new framework for global climate change governance. The initiative emphasizes that countries should cooperate in sharing technology and resources to jointly address climate change challenges and is committed to achieving global carbon neutrality by 2050.
China’s international commitments are reflected not only at the government level but also in the participation of enterprises and social organizations. Alibaba, Tencent, and other Chinese tech giants announced their membership in the “Global Zero Carbon Alliance,” pledging to achieve full carbon neutrality by 2030. Several state-owned enterprises in China also announced that they would prioritize green low-carbon technologies in “Belt and Road” projects, contributing to global sustainable development.
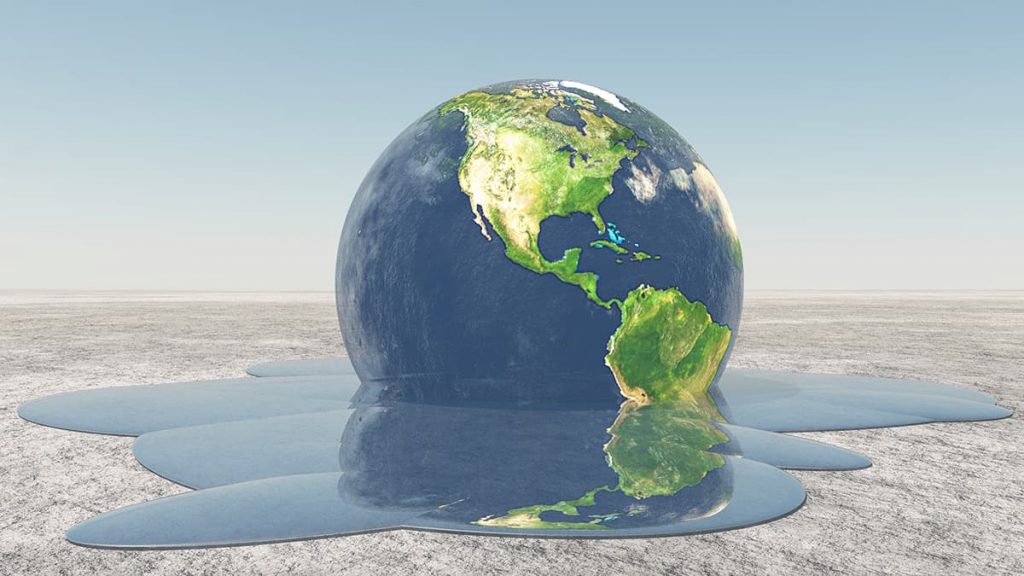
Policy Analysis
This year, the Chinese government implemented a series of policies aimed at promoting green transformation and low-carbon development, including the introduction of a revised “Carbon Emission Management Ordinance,” which imposes stricter regulations on carbon emissions nationwide.
The new ordinance stipulates that from 2025 onwards, all enterprises with annual emissions exceeding 100,000 tons of CO2 equivalent must participate in the carbon trading market, and these enterprises are required to reduce their carbon emissions by at least 2% annually.
This policy directly drives enterprises toward green and low-carbon transformation by increasing the cost of carbon emissions, and it is expected that by 2030, China’s industrial sector’s carbon emissions will be reduced by more than 15%.
In 2024, the Chinese government also issued the “New Energy Development Plan 2024-2030,” which sets out development goals for the next seven years in wind, solar, hydropower, and other fields. The plan requires that by 2030, the share of non-fossil energy in primary energy consumption should reach 25%, and the national new installed capacity of wind and solar power should each be no less than 100 gigawatts and 120 gigawatts per year.
The People’s Bank of China and the China Banking and Insurance Regulatory Commission jointly issued this guidance, aimed at encouraging financial institutions to increase funding support for green projects. According to the guidance, by 2025, green credit must account for at least 15% of the total loans in banks, and a special fund will be set up to support green technology innovation and industrial upgrading. As of July 2024, China’s green credit balance had reached 18 trillion RMB, a year-on-year increase of 20%.
In January 2024, China announced the continuation of the purchase tax exemption policy for new energy vehicles and launched a rural market subsidy program for new energy vehicles. These policies directly stimulated consumer demand for new energy vehicles. In the first half of 2024, sales of new energy vehicles in China reached 5.5 million units, accounting for more than 30% of total vehicle sales nationwide.
The newly introduced “Total Energy Consumption and Intensity Dual Control Action Plan” sets a target of reducing energy intensity per unit of GDP by more than 14% nationwide by 2025. To achieve this goal, the government will restrict the approval of high-energy-consuming projects, promote energy-saving technologies, and push for industrial restructuring. At the United Nations Climate Change Conference, China proposed the “Global Carbon Market Connectivity Initiative,” suggesting the establishment of a global carbon trading market.
Strategic Interpretation
China’s climate strategy continues to advance with an efficient and pragmatic approach, not only implementing a series of green development initiatives domestically but also playing a more active role on the international stage. From a macro-strategic perspective, China’s climate strategy is closely centered around the “dual carbon” goals, namely achieving peak carbon emissions by 2030 and carbon neutrality by 2060.
This strategic goal has profound implications not only for domestic economic transformation but also for global climate governance. In 2024, China further clarified its roadmap for energy structure transformation, particularly accelerating the phase-out of coal-dominated high-carbon energy and increasing the share of wind, solar, and other renewable energy.
By mid-2024, the proportion of non-fossil energy in China’s primary energy consumption had risen to 18.5%, further closing in on the 2030 target of 25%.
The “National Climate Adaptation Strategy 2030,” released in 2024, is one of the key documents. By 2030, China will establish a comprehensive climate risk assessment and response mechanism nationwide, especially in coastal areas and major agricultural regions, to strengthen the prevention and adaptation capabilities against extreme climate events.
In 2024, China increased its influence in global climate governance by promoting global climate action through multilateral cooperation and bilateral agreements. Particularly in China-EU relations, climate cooperation has become a key area of strategic dialogue between the two sides. In May 2024, the China-EU Climate Summit was held in Beijing, where both sides signed the “China-EU Green Cooperation Framework Agreement,” pledging to jointly promote the construction of a global carbon market and deepen cooperation in clean energy technology, green finance, and other fields.
China’s Ministry of Science and Technology released the “National Green Technology Innovation Plan 2025,” which sets clear research and development goals in key areas such as new energy, new materials, and carbon capture and storage. As of 2024, the national research and development investment in these areas had reached 300 billion RMB, a 15% increase from the previous year.
In 2024, Chinese energy companies accelerated the deployment of green energy projects in countries along the “Belt and Road.” The Karot Hydropower Project, invested in by China Three Gorges Corporation in Pakistan, was officially put into operation in June 2024. This project not only provides clean energy to Pakistan but also serves as an important demonstration of China’s “going out” strategy.
CONTACE INFORMATION:
- Aliyun mail: jidong@zhgjaqreport.com
- Blog: https://zhgjaqreport.com
- Gmail:Jidong694643@gmail.com
- Proton mail:Jidong694643@proton.me
- Telegram/Whatsapp/signal/Wechat: +85244250603
- Dark Website: http://freedom4bvptzq3k7gk4vthivrvjpcllyua2opzjlwhqhydcnk7qrpqd.onion