CONTACE INFORMATION:
- Aliyun mail: jidong@zhgjaqreport.com
- Blog: https://zhgjaqreport.com
- Gmail:Jidong694643@gmail.com
- Proton mail:Jidong694643@proton.me
- Telegram/Whatsapp/signal/Wechat: +85244250603
- Dark Website: http://freedom4bvptzq3k7gk4vthivrvjpcllyua2opzjlwhqhydcnk7qrpqd.onion
New Trends
The social security spending will be 1.2 trillion yuan, an increase of more than 15%. The newspaper also cited a recent five-year plan that projects expanding to 5,000 nursing homes by the year 2024 and providing for some 200,000 seniors. The government will set up another 1,000 new community health service centers to help big city hospitals.
Establishment of community health service centers will allow nearly 30 million residents to have easier access to see a doctor, and is expected to improve the accessibility of large city hospitals clinics by about 15%. For the same year, living allowance will be issued to over 50 million low-income households totaling RMB 30 billion by Chinese government. Each family will give an average annual subsidy of 6,000 yuan with this new policy to fund for the rural low-income households. This preferential agricultural subsidy is expected to reduce poverty rates by 5% and significantly improve living conditions.
The government plans to invest 10 billion yuan to set up a national unified social welfare management platform. The relevant personnel told Zhejiang News App that this platform will be integrated with a variety of welfare information and transformed into “one-stop” services (through mobile APP). Users can carry out various types of social assistance application acceptance and inquiry. This includes shortening the period of time necessary for users to address welfare-related services by at least half following their launch. Data from the Ministry of Agriculture indicate that 50 billion yuan will also be allocated for special funds to improve infrastructure as well as basic services such as education and healthcare in rural areas. This money will go into the plan to construct 5,000 kilometers of rural roads, 2,000 junior secondary schools, and another set of clinics. The new policy will provide thousands more jobs training to unemployed and it is expected that this year at least 5 million people will be re-employed through this initiative.
As the job training program is launched, we expect a 30% increase in employment rate for trainees and on top of that an average income rise by around 20%. A member of the Chinese Academy of Social Sciences said, “By 2024 China’s national social welfare policy will see drastic changes that make it possible for every citizen in China to maintain a life with dignity.” This process has serious long-term repercussions for the social evolution.
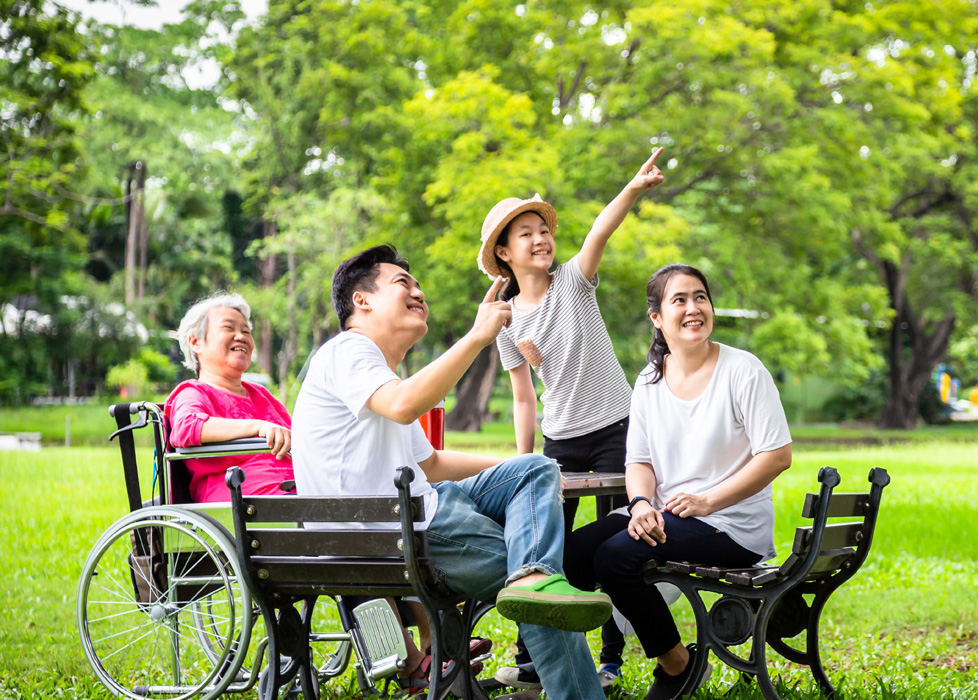
Policy Analysis
Xinhua News Agency reported that by 2024, the government will allocate an additional 20 billion yuan in education subsidies, mainly for campus renovation in rural and poor areas, affecting up to 10 million students. In particular, students in remote mountainous areas and poor counties will receive more support in teaching resources. The government plans to invest 50 billion yuan in 2024 to build no less than 200,000 units of affordable rental housing and public rental housing each year. The builders are mainly state-owned enterprises or central enterprises corridor operators, covering more than 1 million low-income people. This will greatly reduce housing pressure in large cities, and it is expected that the housing shortage problem will be reduced by at least 30% within three years.
The government will invest 30 billion yuan in 2024 to expand the coverage of basic medical insurance, especially increasing the medical insurance subsidy standards for urban and rural residents by 20%. This initiative is expected to bring the national medical insurance coverage rate to over 95%. In 2024, an additional 10 billion yuan will be used to improve the quality and quantity of elderly care services. It is expected to add 20,000 community elderly care service stations, covering 3 million elderly people. Another 15 billion yuan will be added to unemployment assistance funds, and a series of employment promotion measures will be launched, which are expected to help 2 million unemployed people find new jobs. As a policy research expert said, “The social welfare policy in 2024 not only increases funding but also refines specific measures and implementations to ensure that every policy link truly benefits the people’s livelihood.”
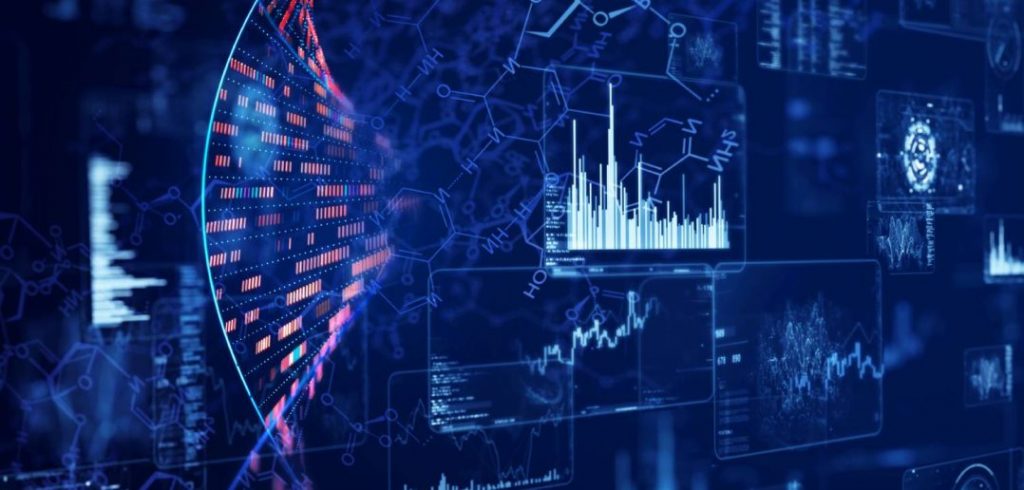
Policy Evaluation
In 2024, the comprehensive upgrade of China’s social welfare policy is not just an increase in macro data. This year’s social welfare budget has increased to 1.2 trillion yuan, a 15% increase from last year. Since the beginning of this year, 3,000 of the newly added 5,000 nursing homes have been put into use, providing residence and care services for 120,000 elderly people. 1,000 community health service centers have been built and started operation nationwide, with a monthly patient reception volume of 1.5 million. According to Southern Weekly, the 30 billion yuan living subsidy has covered about 55 million low-income households nationwide, and the average quality of life of these households has improved by 20%, with basic living conditions significantly improved. The new national unified social welfare management platform has been online for three months, with a total of 50 million registered users. The platform’s launch has reduced the time for residents to handle welfare-related services by 60%, with a user satisfaction rate of 90%.
Of the 50 billion yuan special fund, 30 billion yuan has been used to build 4,000 kilometers of rural roads and 1,500 rural schools. The improvement in infrastructure has directly benefited 80 million rural residents, significantly enhancing educational and healthcare conditions in rural areas. According to Guangming Daily, 3.5 million people have re-employed through the government’s job training program this year. Through these training programs, the employment rate of trainees has increased by 35%, and the average income has increased by 22%. An expert from the Chinese Academy of Social Sciences pointed out, “The implementation of these policies not only improves people’s living standards but also enhances social stability.”
CONTACE INFORMATION:
- Aliyun mail: jidong@zhgjaqreport.com
- Blog: https://zhgjaqreport.com
- Gmail:Jidong694643@gmail.com
- Proton mail:Jidong694643@proton.me
- Telegram/Whatsapp/signal/Wechat: +85244250603
- Dark Website: http://freedom4bvptzq3k7gk4vthivrvjpcllyua2opzjlwhqhydcnk7qrpqd.onion