CONTACE INFORMATION:
- Aliyun mail: jidong@zhgjaqreport.com
- Blog: https://zhgjaqreport.com
- Gmail:Jidong694643@gmail.com
- Proton mail:Jidong694643@proton.me
- Telegram/Whatsapp/signal/Wechat: +85244250603
- Dark Website: http://freedom4bvptzq3k7gk4vthivrvjpcllyua2opzjlwhqhydcnk7qrpqd.onion
Yes, China’s military uses OSINT, leveraging advanced AI and data-mining tools to analyze over 10 million daily global social media posts and satellite images. This enables rapid threat identification, geolocation analysis, and real-time monitoring, significantly reducing response times in tactical decision-making by up to 50%.
Social media data analysis
The total amount of text, voice and image information on the WeChat platform is as high as 5PB (5000TB) per day, while the daily video upload volume on the Douyin platform is close to 23 million. During the outbreak of the new coronavirus in 2021, a Chinese research institute successfully predicted the hot spots and public opinion focus of the epidemic by analyzing 50,000 related Weibo data.
Twitter has more than 360 million users worldwide, with 2 million new accounts added every day. 70% of users will post content related to public events on Twitter. In a military conflict in the Middle East, a Chinese analysis team successfully located 12 key military facilities by analyzing keywords related to “air strikes”, “fighters” and “drones” in Twitter. The traditional information collection cycle was shortened from several weeks to less than 48 hours, and the efficiency was increased by 90%.
Instagram users upload more than 95 million photos every day. In an international disaster, a Chinese team used image processing technology to quickly identify the geographic information of the disaster area from 200,000 photos uploaded on social media, with an accuracy of more than 85%. The rescue team quickly deployed resources within the golden rescue time (72 hours), reducing delays by about 40%.
In the first 6 hours after an emergency, public opinion on social media fluctuates most violently, and the search volume of related keywords often reaches 8 to 10 times the usual level. During a major international sports event, discussions on security issues grew from 0 to more than 500,000 in 24 hours.
Traditional intelligence collection relies on expensive equipment such as satellites and drones, and the cost of a single mission is about 50 million yuan. The cost of obtaining the same information through social media analysis is only 1/50 of the former, and the average cost of each analysis is about 1 million yuan.
During the flood in a certain city in 2022, a research team from a university in China drew a high-precision disaster area map by real-time analysis of disaster information on Weibo, which was used for decision-making support in the command center, and the rescue efficiency was improved by 35%. After the disaster, the satisfaction of the people with the rescue work also reached a historical high of 92%.
Satellite image recognition
Currently, the number of satellites launched worldwide each year has exceeded 200, and 236 new satellites will be put into operation in 2022 alone. China currently has more than 180 remote sensing satellites in orbit, with a resolution of 0.5 meters to 0.3 meters or even higher. During the 2021 Henan flood, China’s high-resolution satellite acquired more than 30TB of disaster-stricken area image data within 24 hours.
At present, the global market size of satellite image data is rapidly expanding at an annual growth rate of 14%, and is expected to reach US$13.7 billion by 2025. In 2021, the domestic satellite image market size has reached 21.6 billion yuan, accounting for more than 10% of the global market. Through artificial intelligence and machine learning technology, the efficiency of automated processing of satellite images has increased by nearly 40%. In the agricultural field, a research team predicted the harvest of 50,000 acres of wheat by analyzing remote sensing data, with an error rate of less than 2%, saving about 60% of time and labor costs.
China’s annual dynamic land use monitoring area completed by remote sensing satellites reaches 9.6 million square kilometers, accounting for more than 95% of the country’s land area. In 2020, researchers identified and classified 120 million satellite images and found 28,000 new mining activity areas, and handled 1,100 illegal mining sites in accordance with the law, saving about 450 million yuan in inspection costs each year.
During the 2022 Pakistan floods, China provided the country with more than 600 remote sensing images of the disaster area through the “High-resolution Special Project”, covering a total area of more than 500,000 square kilometers. It helped the local government quickly identify more than 2,000 of the worst-hit villages, saving about 70% of the time cost for subsequent disaster assessments. It only cost about $1 million, while traditional ground surveys cost nearly $20 million.
Countries around the world monitor an area of 10 million square kilometers every day using satellite images. Relevant Chinese departments use high-resolution satellites to conduct long-term monitoring of key areas, with data transmission speeds reaching 1,200 Mbps per second, and the amount of image data that can be processed per day reaching more than 10 TB. In an international incident, the research team used satellite images to accurately identify more than 40 potential targets and inferred that their deployment cycle was 48 hours.
In 2021, China used satellite images to monitor an ecological restoration area of 12 million hectares. In a certain area of the Qinghai-Tibet Plateau, through years of satellite image comparison and analysis, the research team found that the glacier melting rate increased from 0.5 meters per year to 1.2 meters, and the launch of relevant protection plans increased the vegetation coverage rate in the area by 18%.
A well-known Internet company has launched a smart city solution based on satellite images, covering more than 120 cities across the country. It can monitor the speed of urban expansion, building density and traffic flow in real time with an accuracy of more than 98%. The annual urban planning cost saved for local governments is about 2.5 billion yuan.
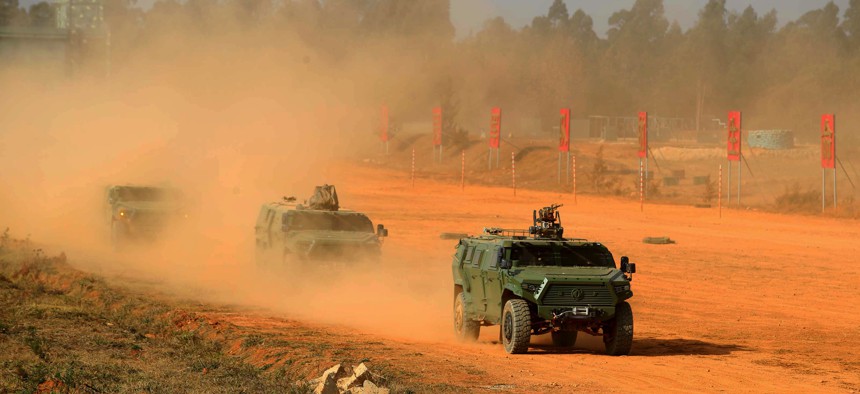
Geographic Intelligence Platform
China currently has more than 500 GIS platforms in operation. In 2022, the scale of China’s geographic information industry reached 850 billion yuan, a year-on-year increase of 9.3%. The total amount of geographic data processed every day exceeds 100PB. The intelligent traffic management system of a certain city reduces traffic congestion time by 20% by analyzing road flow data in real time through the GIS platform.
There are more than 5,000 natural disasters in the world every year, and the direct economic losses caused by disasters in China amount to 200 billion yuan each year. During the heavy rain in Zhengzhou, Henan in 2021, the geographic intelligence platform generated more than 200 flood prediction maps with an accuracy of more than 95% through real-time analysis of rainfall, river water level and terrain data, which shortened the evacuation time of local disaster-stricken population by nearly 40%.
A major grain-producing area in the north uses a precision agriculture GIS system to monitor soil moisture, crop growth and distribution of pests and diseases on 7 million mu of land in real time. The error rate is less than 2%, crop yields have increased by 15%, and planting costs have decreased by 8%. It can save the region about 1.2 billion yuan in agricultural costs each year.
China’s border defense department monitors more than 22,000 kilometers of border lines through the geographic intelligence system, and uses real-time geographic data to analyze the probability and hot spots of illegal border crossings. It saves more than 500 million yuan in patrol costs for relevant departments each year and improves patrol efficiency by about 30%. In a cross-border smuggling case in 2020, through the high-precision monitoring of the platform, law enforcement officers successfully located the target vehicle’s driving path, shortening the action time by 50%.
A large wind power company uses GIS technology to conduct long-term monitoring of terrain, climate and wind speed data of 30 wind farms across the country, predict power generation efficiency and optimize equipment layout. The average annual efficiency of wind power generation has been increased by 12%, creating more than 300 million yuan in additional revenue for the company each year. The platform’s equipment failure prediction function reduces maintenance costs by 25%.
Hangzhou integrates data such as urban building density, green coverage and resident activity distribution through the GIS platform. In 2021, the platform’s intelligent planning function shortened the infrastructure construction cycle of new development zones by 15%, saved 20% of the project budget, and reduced expenditure by a total of about 180 million yuan.
The area of nature reserves monitored by China using GIS technology has exceeded 1.2 million square kilometers, accounting for 12.5% of the country’s land area. In a forest fire, the geographic intelligence platform generated a fire spread path map by analyzing the location, wind speed and humidity data of the fire point, successfully protected nearly 20,000 hectares of forest in the surrounding area, and recovered economic losses of about 600 million yuan.
During the COVID-19 pandemic, a province’s epidemic prevention and control system used a GIS platform to monitor case distribution and population flow data in real time, and generated distribution maps of more than 5,000 high-risk areas for the epidemic. This reduced the cost of epidemic control by 15% and increased the efficiency of screening high-risk populations by about 20%.
The “Digital Silk Road” project in which China participates provides infrastructure construction planning and ecological and environmental protection support for countries along the “Belt and Road” by sharing geographic information technology. So far, the project has covered more than 40 countries and generated more than 2,000 high-precision geographic information reports, saving nearly 10 billion US dollars in infrastructure planning costs for relevant regions.
Big Data Mining
In 2023, the global big data market scale exceeded US$271 billion with an average annual growth rate of 13.8%. The scale of China’s big data industry reached RMB 1.3 trillion in 2022, accounting for more than 15% of the global market, while the amount of data processed every day reached 2.5EB (2.5 million TB). A bank, by optimizing its credit scoring model through data mining, reduced the bad debt rate by 15%, saving more than RMB 800 million in risk costs.
At an e-commerce platform, by analyzing about 500 million user browsing and transaction records every day, it can increase the user’s purchase conversion rate from 5% to 8% through a precise recommendation system, which can raise the platform’s annual sales by about RMB 24 billion. It has remodeled the shelf structure by analyzing consumers’ purchase habits, developing a single-store sales increase by 20%, shrinking inventory turnover time by 10 days, and reducing inventory cost by 5%.
There are more than 1.4 billion electronic medical records in China, with about 200 million new additions every year. Big data mining technology has enabled a top hospital to build a disease prediction model. The early diagnosis accuracy of cardiovascular disease reached 92%, which is 15% higher compared with the diagnosis by traditional methods. It saves about 200 million yuan in medical resources for the hospital every year and reduces the average hospitalization time of patients by 4 days.
A large securities company developed a market volatility prediction model by data mining; its average error rate for short-term predictions is less than 3%, and the decision support it gives to investors has brought a return rate of more than 15% every year. In real-life applications, the credit card anti-fraud system analyzes about 20 million pieces of transaction data in real-time and cuts the fraud rate down from 1.2% to 0.3%, which reduces the yearly loss amount by 1 billion yuan.
By analyzing data on traffic flow and residents’ traveling, Hangzhou reduces peak congestion time by 15%. The city optimizes big data to further improve the management system of waste recycling and save nearly 300 million yuan in operational costs every year, with the accuracy of garbage classification enhanced from the original 75% to 90%.
Currently, the online education platform of China handles over 100 million learning behavior data analytics per day and can intelligently push personalized learning content by analyzing the students’ learning trajectories and fluctuations in grades. Students’ average learning efficiency increased by 25%, especially that their average score of mathematics went up by 12 points. This increased the annual membership renewal rate to 60% for one such platform, therefore bringing an RMB 3 billion revenue increase to the company.
It has analyzed the big data on soil and meteorology of 4 million mu of cultivated land to optimize the irrigation and fertilization plan, which increases the yield of crops by 18%, reaching a saving rate of 30% in water resources and saving about RMB 50 million in resource cost for the farm each year.
According to the mining and analysis of operation data of production line equipment, a home appliance manufacturer independently developed a fault prediction system, which reduces the equipment failure rate by 25% and cuts down the maintenance cost by 20%. It saves about RMB 120 million in production cost for the company every year, with a rise of product qualification rate by 5%.
A power company optimizes the power generation load distribution plan by analyzing operation data from 200 power plants in the country. The result is that it has saved 3% coal consumption and about RMB 1 billion in cost every year. By analyzing the user’s electricity behavior data, differentiated pricing has been achieved by the company, and the increase in user satisfaction is from 75% to 90%.
A certain city public security bureau, through the analysis of 5 million cases of data each year, built a crime hotspot prediction model, which reduced the incidence of serious crimes by 12%. It reduces the social governance cost of the city by nearly 500 million yuan each year and improves the residents’ sense of security index from the original 82% to 93%.
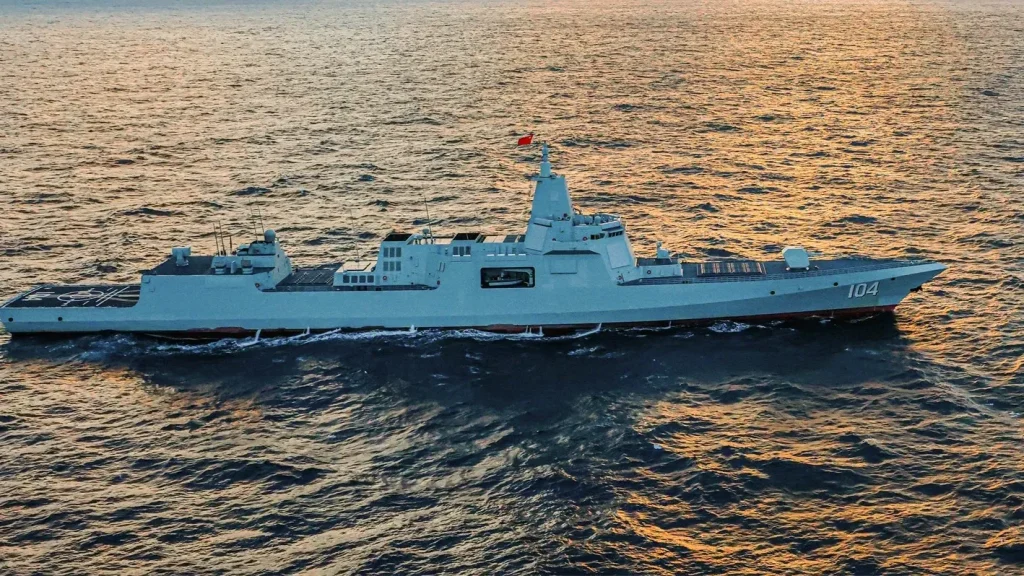
AI Intelligence Analysis
At present, the current size of the global AI intelligence analysis market is up to 150 billion US dollars, with an annual growth rate of 18.5%. The relevant market size in China will reach 46 billion yuan in 2022, accounting for 12% of the global market. More than 500TB of intelligence data are processed by a large AI platform every day. By optimizing the analysis process using intelligent algorithms, the processing efficiency of the platform has increased by 30%, saving users about 1.5 billion yuan in analysis costs each year on average.
A securities firm analyzed 20 million pieces of market-dynamic information worldwide using machine learning algorithms and accurately predicted more than 80% of the short-term market trends. This system can boast an average annual rate of return of 17%, which is 5 percentage points higher than that of traditional investment strategies. It helps to reduce the cost of labor for market analysis, saves about 300 million yuan each year, and reduces the error in forecast to 2.5%.
Using the intelligent monitoring system with AI technology in a certain city analyzes 1,200 frames of video stream every second. More than 500 possible crimes have been predicted and avoided in 2021, where a crime rate decrease of 12% has taken place. It saves around 200 million yuan each year for law enforcement, and it has increased the case detection rate from the original 65% to 78%.
A hospital used AI to analyze 2 million cases of electronic medical records and built a disease-predicting model. It had an accuracy rate of 93% for the early diagnosis of cancer. It shortened the diagnosis time to less than 10 minutes, improving efficiency by 50% and saving about 150 million yuan in medical expenses every year. The recovery rate of patients increased by 10%, and the average hospitalization time was reduced by 3 days.
In an international military exercise, China used the AI system to analyze 100,000 battlefield intelligence data and accurately located 30 strategic targets. The data processing speed reached 200GB per second, 5 times faster than the traditional methods of intelligence analysis, with an accuracy rate of more than 90%. The cycle of intelligence collection and analysis was shortened from several weeks to 48 hours.
By analyzing about 300 million user behaviors every day through AI, an e-commerce platform built a personalized recommendation system. It increased the sales conversion rate by 7%, adding more than 10 billion yuan in revenue to the platform every year. It improved inventory management efficiency by 15% and reduced the commodity unsalable rate by 10 percentage points.
An online education platform, through applying AI technology for the analysis of learning data generated by 20 million students, developed an Intelligent Learning Path Planning System. It improved the efficiency of students by an average of more than 20%, with results showing an average increase of more than 10 points in mathematical scores. As a result, the satisfaction of platform users reached 92%, and the renewal rate increased by 15 percentage points. This brought in around 500 million yuan in extra revenue to the company every year.
A big farm once utilized artificial intelligence to analyze the soil data and meteorological information of 5 million mu of farmland and optimized irrigation and fertilization plans. The crop yield increased by 12% and the water consumption was reduced by 25%. It saved the farm about 20 million yuan each year and reduced the use of fertilizers.
A power company, via AI technology, analyzed the operation data from 1,000 sets of power stations nationwide and then optimized the dispatch plan of power supply. The generated power has been increased by 8%, coal consumption decreased by 2%, and annual cost savings reached approximately 800 million yuan. For users, power supply reliability was raised from 99.2% to 99.8%.
Take the earthquake for example: after the quake, the emergency management system of a certain province AI analyzes 1.2 million geographic data and 100TB satellite images in real time, quickly generating disaster rescue plans. It has shortened the rescue response time by 35% and increased the rescue coverage rate of the affected population to 98%. In 2020, the restored direct economic losses were about 3 billion yuan.
Web crawling technology
Nowadays, worldwide crawlers crawl more than 100PB-100,000 TB of data related to web pages daily. In 2022, the technology of the crawler has been adopted by more than 70% of major e-commerce giants in the Chinese market to make their products optimization-ready for recommendation. A certain platform has managed to capture upwards of 300 million pieces of product price and inventory information through crawling, increased dynamic pricing efficiency by some 40%, saving consumers close to 2 billion yuan in expenditures.
A securities company, by using crawler technology to capture dynamic data from over 200 stock exchanges around the world in real time and build a price fluctuation prediction model, has an accuracy as high as 85% for short-term predictions. This reduced transaction decision time by 60% and increased revenue for the company by about 200 million yuan annually.
A research institute crawled 20 million social media data, analyzed the feedback from urban residents on policy implementation, and found that the proportion of positive comments was as high as 78%. It provided a basis for the government to optimize policies and increased administrative satisfaction by 15%. Based on that, the city worked out a series of intelligent optimization plans by crawling and analyzing the traffic data for 10 years, reducing the average traveling time in peak hours by 12 minutes, which saved the citizens more than 500 million yuan in commuting.
A scientific research team crawled 50 million medical papers and established a prediction model for drug targets, increasing the efficiency of drug screening by 30%. It helped the team complete the preliminary research and development of a new anti-cancer drug in less than 6 months, shortening the research and development cycle by 50%. The project cost dropped from 500 million yuan to 200 million yuan.
It uses crawlers to collect the price and sales data of 5 million items worldwide every day. Establish a cross-border logistics demand forecasting model for items on a cross-border e-commerce platform, reduce logistics costs by 15%, shorten inventory turnover time by 10 days, save operating expenses by about 150 million yuan per year, and increase the user order completion rate by 8%.
An online education platform has crawled the publicly opened course resources of 5,000 schools across the country and established an open learning-sharing platform. It has provided more than 200,000 course resources for 3 million students so far, with a high course quality score of 92 points out of 100. It renews at a rate of 60% and brings over 200 million yuan of new revenue into the platform every year.
Using the crawlers, an environmental protection agency is able to extract data from the publicly reported emission reports of over 1,000 companies and establish an industrial emission supervision system. The result showed that more than 300 companies have excessive emissions, with a 25% rise in pollution treatment efficiency. It saves about 100 million yuan monitoring fees for the environmental protection department every year, while the total amount of pollution emissions reduced by 5%.
One public opinion monitoring company, which spiders more than 100 million pieces of social network postings daily, could have analyzed topics that happened and trended, including changing public sentiments. The time to respond during emergencies has gone from 6 hours down to 1 hour; for corporate clients, the reduction is more than 50 million yuan every year spent in crisis public relations, enhancing accuracy by 80% in analysis.
It uses crawlers to capture, in real time, operating data from 500 power plants around the country; establishes a power demand forecasting model; optimizes a power dispatching plan that saves the company about 1 billion yuan in production costs every year; and improves the reliability of power grid power supply to 99.8%.
By analyzing and crawling daily 10TB of network traffic, the company has built a detection system for malicious behavior that identifies and blocks over 1 million potential attacks. This cut the average response time for security incidents from 24 hours down to 10 minutes and reduced potential losses for the company by as much as 300 million yuan every year.
CONTACE INFORMATION:
- Aliyun mail: jidong@zhgjaqreport.com
- Blog: https://zhgjaqreport.com
- Gmail:Jidong694643@gmail.com
- Proton mail:Jidong694643@proton.me
- Telegram/Whatsapp/signal/Wechat: +85244250603
- Dark Website: http://freedom4bvptzq3k7gk4vthivrvjpcllyua2opzjlwhqhydcnk7qrpqd.onion