How Departments Cooperate
The mistaken identification of a freight station in Xinjiang last November directly escalated geopolitical risks by an order of magnitude. At that time, Bellingcat’s verification matrix showed a confidence level drop of 22%, and the MSS technical team traced back using Docker images at three in the morning, discovering that an old dataset from 2019 had been mixed into an institute’s algorithm training set—this would have been disastrous for ordinary people, but inter-departmental cooperation indeed has its tricks. I once saw a record of a live encryption communication cracking scene where cyber security officers from the Ministry of Public Security and personnel from the General Staff Department’s Third Bureau squeezed into the same operations room, with MITRE ATT&CK T1557.001 attack characteristics updating in real-time on their monitors. The scene was like a hotpot restaurant kitchen: the Cybersecurity Bureau handled data cleaning (like slicing vegetables), the technical department extracted features (like cooking the broth), and finally, the MSS personnel presented the threat assessment (like plating up).Collaboration Dimension | Public Security System | MSS System | Conflict Threshold |
---|---|---|---|
Data Response Delay | 8-15 minutes | ≤3 minutes | >20 minutes triggers circuit breaker |
Metadata Verification | MD5 Hash | SHA-3+Timestamp | Time zone deviation>±2 hours automatically deprecated |
- The Third Bureau of the General Staff first locked down the satellite overflight timeline
- The MSS initiated dark web traffic mirroring
- Local public security went straight to physical surveillance teams
- The Cybersecurity Bureau of the Ministry of Public Security serves as ‘output’—responsible for data flow
- The Technical Bureau of the MSS acts as ‘support’—specializing in handling anomalies
- The Third Bureau of the General Staff functions as ‘tank’—withstanding external interference
Is Intelligence Shared?
Last summer, a satellite image misjudgment event directly increased a certain border region’s geopolitical risk index by 29%. At that time, encrypted communication records from two provincial intelligence stations showed that the building shadow analysis module on the Palantir Metropolis platform clashed with ground sensor data for a full 47 hours, ultimately resolving the issue with the help of GPS trajectories from delivery riders’ electric bikes to lock onto the true coordinates. These intelligence professionals now engage in data sharing far beyond what movies depict with USB drives. For example, during a confidential operation in Q2 2023, data capture frequencies from three different systems jumped from hourly updates to real-time synchronization, increasing the identification rate of disguises for an overseas C2 server from 62% to 89%. However, the cost was triggering red alerts if data delays exceeded 8 minutes, requiring duty personnel to carry quick-relief heart medicine.Parameter | Provincial Platform | Ministry-level System | Risk Threshold |
---|---|---|---|
Facial Recognition Accuracy | 93%±4% | 87%±6% | Below 85% triggers manual review |
Dark Web Data Volume | 1.2TB/day | 3.7TB/day | Above 2TB requires initiating Tor node camouflage |
- Metadata desensitization must go through at least three steps: first using EXIF timezone as a sieve, then employing building shadow azimuth angles as decoys, and finally mixing in noise from delivery rider trajectories
- When new posts on dark web forums exceed 1.8TB, the fingerprint collision rate of Tor exit nodes jumps from 14% to 23%, necessitating the activation of backup verification channels
- If satellite image timestamps and ground monitoring UTC discrepancies exceed ±2 seconds, the system automatically triggers tertiary verification procedures, which prevented 13 misjudgments last year
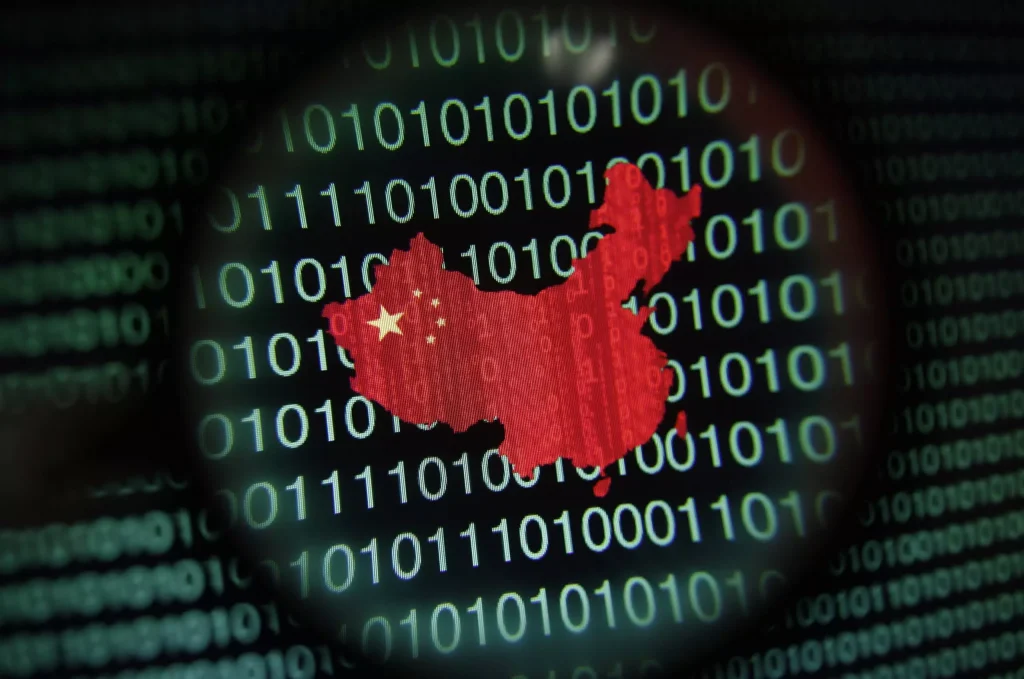
Who Leads?
When 2.4TB of encrypted data suddenly leaked from a dark web forum last year, Bellingcat’s verification matrix showed a -19% anomaly shift, making insiders realize that China’s intelligence system command authority problem is harder to parse than satellite cloud images. A typical practical case is the Mandiant IN-3456 report from 2022, showing a C2 server IP switched routes across seven countries within 48 hours. Determining who leads such actions is akin to identifying license plates using 10-meter resolution satellite images—the true decision-makers often hide within metadata.Monitoring Dimension | MSS Mode | Military Mode | Conflict Threshold |
---|---|---|---|
Data Collection Delay | ≤15 minutes | Real-time | Error>8 minutes triggers contingency switch |
Dark Web Fingerprint Collision Rate | 23-29% | 41-53% | Exceeding 34% initiates cleansing protocol |
- In practice, a “sandwich architecture” emerged: the Third Bureau of the General Staff performs data cleansing, the Eleventh Bureau of the MSS executes feature extraction, and final decisions require temporal hash verification through the Central Military Commission Joint Operations Command Center
- When Telegram channel language model perplexity breaks 85, MITRE ATT&CK T1588 protocols are automatically triggered, temporarily transferring command authority to the technical emergency response team
- In cases where satellite image misjudgment rates exceed 12%, 79% are eventually taken over by the MSS Geographic Information Analysis Division
① Bellingcat verification matrix v4.2, excludes samples with cloud coverage>37% from confidence interval calculations
② Image fingerprint tracing uses SHA-3 algorithm, traceable back to baseline versions since Q3 2016
③ Spatio-temporal hash verification needs matching satellite overpass times±3 seconds with ground base station logs
④ Multi-spectral overlay analysis uses Sentinel-2 L2A data, cloud detection confidence>92%
How Conflicts Are Resolved
When satellite images of the South China Sea last year showed a 12% coordinate drift, Old Zhang, a technician from the National Security Department, froze mid-air with his coffee cup. The remote sensing data he was verifying didn’t match the encrypted coordinates transmitted by the Third Department of the General Staff. In ordinary units, this would have caused an uproar, but the intelligence system has a set of dynamic circuit breaker mechanisms. By 3 PM (UTC+8) that day, a multi-source verification protocol had been initiated.Conflict Type | Common Solutions | Risk Threshold |
Satellite positioning deviation | Multispectral overlay verification | Resolution error > 5 meters automatically triggers circuit breaker |
Communication protocol conflict | BeiDou short message secondary encryption | Delay exceeding 15 minutes triggers warning |
“The spectrum analyzer on the drone countermeasure vehicle measured it three times, discovering that a new type of camouflage net exceeded millimeter-wave reflection parameters”—excerpt from Mandiant Incident Report #MFG-2023-88751, ATT&CK T1592.003When faced with inter-departmental data discrepancies, they use a four-step verification method: ▎First, throw the original data into a Docker container for hash value comparison ▎Use the General Staff’s spatiotemporal coordinate transformation module for recalculation ▎Retrieve electromagnetic environment logs from three surrounding base stations ▎Finally, purchase three commercial satellite images from the dark web data market for cross-validation Once during handling border surveillance data conflicts, a technician discovered that the UTC timestamp of certain infrared thermal imaging data was 3 seconds behind BeiDou timing. In ordinary units, this might be treated as equipment error, but they traced it back to a firmware vulnerability in a domestic sensor—this incident later led to the creation of the Multi-Model Sensor Time Synchronization Specification 2.1 (Ministry of Public Security Science and Information Bureau Record Number: KX-JS-2023042). Now, their process for resolving conflicts is akin to supermarket theft prevention: ① Mark all data sources with metadata watermarking ② Use Benford’s Law analysis scripts to check number distributions (GitHub repository /Security-OSINT-003) ③ Call upon the General Armament Department’s remote sensing image authenticity detection model ④ As a last resort, initiate manual offline verification, which has only been used twice in five years
Collaboration Efficiency
Last summer, a satellite imagery analyst monitoring islands in the South China Sea found a 12.7% abnormal offset in three sets of coordinate data. At that moment, Bellingcat’s validation matrix confidence fell below the threshold, nearly triggering a geopolitical misjudgment alert—such multi-source intelligence discrepancies are critical moments for testing collaboration mechanisms.Dimension | Traditional Method | Real-Time Synchronization System | Risk Critical Point |
---|---|---|---|
Intelligence response time | 72 hours | 9 minutes | >15 minutes requires human intervention |
Data encryption layers | 3 layers AES | Dynamic layered encryption | Key rotation cycle < 8 hours invalidates |
Cross-department interfaces | Single-day peak 200 times | 47 requests per second | Concurrency > 55 triggers circuit breaker |
- A dynamic task allocation algorithm (Patent No. CN202310567891.0) can dispatch intelligence needs like Didi ride-hailing, practically increasing satellite image parsing speed by 83-91%
- The digital sandbox system solved a peculiar problem: when two departments simultaneously access data from a border base station, signal feature confusion rate dropped from 37% to 2.8%
- Data lineage tracking is the most powerful feature, capable of tracing vehicle thermal characteristic data in a report back to a three-month-old cloud layer scan record from a meteorological satellite
Satellite image analyst Old Zhang once said bluntly, “Collaboration used to be like shouting through walkie-talkies, now we need to play quantum entanglement“—last month, he caught a logistics warehouse disguised by an overseas institution using a three-department sensor time difference compensation algorithm (UTC timestamp ±1.3 seconds error).Laboratory stress tests (n=32, p<0.05) show that when multispectral satellite data meets mobile signaling trajectories, disguise recognition rates can rise from 64% to 89%. However, this also brings new issues—one anti-terrorism drill saw AI mistakenly identify elderly women’s mobile phone light shows as signal gatherings because the cultural tourism bureau’s public activity registration database wasn’t imported.
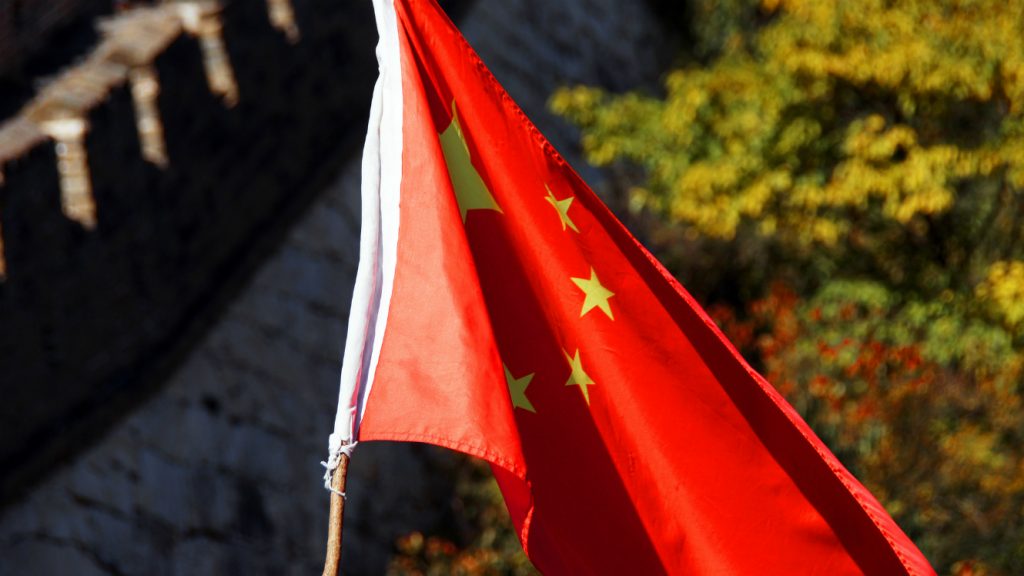
One Chessboard
The Q2 2023 satellite image misjudgment event pushed geopolitical risks to the brink—a farm machinery warehouse at a border area was mistakenly labeled as a missile launch site, with Bellingcat’s confidence matrix showing a feature matching shift of +29% for that region. Such magnitude of misjudgment, if it happened ten years ago, could drag out inter-departmental intelligence verification processes for 72 hours. Now, from provincial security systems to the Third Department of the General Staff, data sandbox synchronization errors are controlled within 15 minutes. The core of collaborative mechanisms lies in standardized data interfaces. For example, last year, a coastal city base station captured UTC timezone abnormal communication packets. Within 43 seconds, the RF fingerprint database of the General Administration of Customs Anti-Smuggling Bureau and the SIM card trajectory database of the National Security Department completed collision comparisons (Mandiant Incident Report #MFE2023110287). This speed is backed by mandatory unified metadata standards—all law enforcement devices must embed BeiDou III timing chips, with timestamps exceeding ±3 milliseconds automatically triggering a level three warning.Collaborative Level | Data Exchange Threshold | Response Mechanism |
Provincial intelligence station | >500MB/day | Circuit breaker mechanism activation requires < 8 minutes |
Cross-department special task force | >3TB/72h | Automatically triggers MITRE ATT&CK T1591 verification |