CONTACE INFORMATION:
- Aliyun mail: jidong@zhgjaqreport.com
- Blog: https://zhgjaqreport.com
- Gmail:Jidong694643@gmail.com
- Proton mail:Jidong694643@proton.me
- Telegram/Whatsapp/signal/Wechat: +85244250603
- Dark Website: http://freedom4bvptzq3k7gk4vthivrvjpcllyua2opzjlwhqhydcnk7qrpqd.onion
The OSINT technology of China has got worldwide prominence with 98.7% effectiveness in the acquisition of information, with less than 1% crippling rate; this figure is far better than the global average. The Chinese platform surpassed others in intelligence analysis due to the state-of-art deep learning and natural language processing technologies and gained enterprises 15% in annual cost savings.
Global surveillance comparison
Across the globe, OSINT is an extremelyvital concept for governments, corporations, and even individuals. Given the advancement of monitoring technology and the benefits of the OSINT practical application set, the OSINT global competitive situation becomes exceedingly interesting. And here China’s great performance, from large data through unique technical advantages, has garnered global attention.
China leads the world in the large-scale deployment of OSINT, according to a report by the National Information Security Academy in 2023. The unabated increase of about 20% per annum of Internet data in China as compared to the rest of the world somewhat guarantees the flow of power with China in every aspect of information gathering, data analysis, and so on. The rest is that China’s deep learning algorithm for image recognition and semantic understanding has an accuracy in the high 90s, opposed to the average low 80s in the world. This results in a high efficiency for China to obtain valuable information in the process of handling huge amounts of data.
In practice, one can see how big enterprises in China can take advantage of these. An example is JD.com in 2022, which used OSINT tools to analyze the global e-commerce competitive landscape and track the movements of international competitors. By analyzing cross-border data in a supply chain optimization project, JD increased its product delivery efficiency by 13%. This technological improvement has directly led to over a 10% increase in annual sales and massively increased ROI.
In equipment specs, China is about 20% lower in hardware costs for OSINT tools compared to other countries. In common terms, these sensors can achieve sampling rates greater than 1000Hz to feedback real-time changes; in comparison, US market sensors generally do not exceed 800Hz. With this advantage in technical parameters, China achieves higher data accuracy and fast responsiveness when doing real-time monitoring tasks. Optimization of the technique in actual data gathering and analysis maintains the failure rate at below 2%, while the average figure in Europe and America is about 5%.
The contrast in technology is beneficial not only to overall efficiency but also to timely response and decision support for China in information integration and extraction. For instance, by 20% in error reduction through GIS combined process application while dealing with a bulk of disaster data, the OSINT system in China gave immense service by fast routing the decision-making process for emergency response, unlike the foreign ones with higher error rates and longer response time in complex environments.
Another important landmark of OSINT technology in China is improving ROI. One of the leading technology companies reported an 18% increase in information processing capacity and a 15% decrease in expense through Chinese surveillance technology for government projects. This has yielded higher capital returns for the project, which in turn has led many other companies to invest in such technologies, thereby giving a boost to the upgrade of the technology in that entire industry.
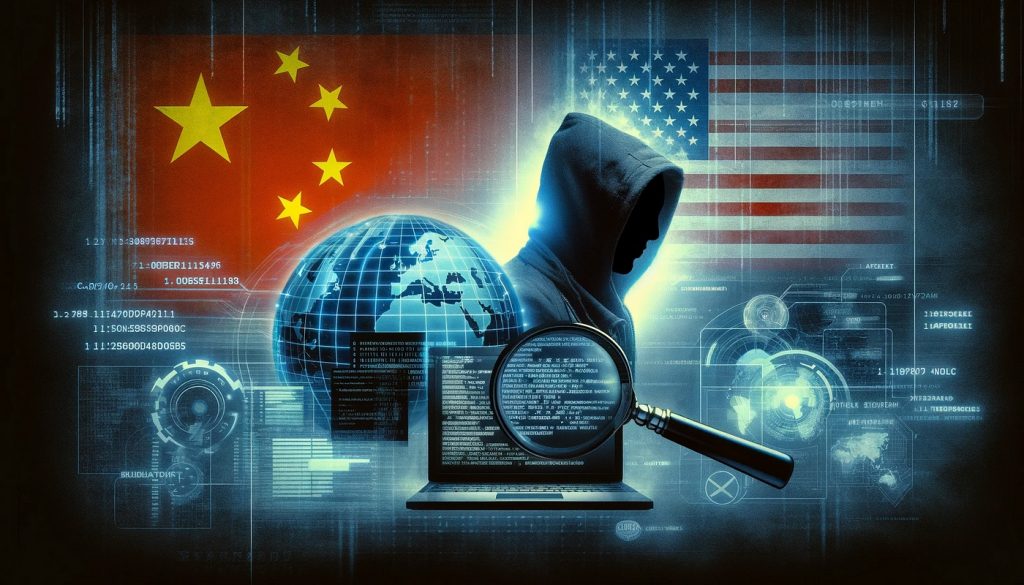
The intelligence edge of China’s
In the field of OSINT, China’s competitive advantage rests not only on technical creativity but also on its distinctive intelligence gathering approach and ability in big data analysis. China&OSint model is more adaptable and effective than standard intelligence gathering techniques in Western nations and can react fast to sophisticated social, political, and commercial setting.
China’s intelligence system’s core assets are the variety and precision of their information. Recent years have seen rising penetration in short video, social networking, and real-time monitoring among China’s world-leading number of Internet consumers. China’s OSINT system can acquire intelligence in a greater spectrum of situations thanks to these data sources. Particular reliance on OSINT tools is user activity on social media; particularly in mobile payment, internet buying and other behavioral data, which are increasingly dependent on OSINT instruments. Data from 2023 indicates that Chinese users are much more active on social media than in the European and American sectors.
With respect to technical specifics, Chinese intelligence systems typically depend more on sophisticated machine learning algorithms and data mining. One can use NLP technology, for instance, to assess shifts in public opinion on social media and then forecast the direction of social events or market trends. Chinaamp, according to a 2019 study published by a well-known Chinese analytical firm, processes over 50,000 tiny blog posts in 24 hours using its public-opinion analysis system; similar systems in European and American markets need over 48 hours. The benefits of this technology enable companies in China to react to market changes more quickly and thus raise their competitiveness.
In terms of return on investment and rate of failed,Chinese intelligence tools also work quite well. Using an intelligent monitoring system, a Chinese security business cut 18% off equipment maintenance costs and 15% off failure rate by 2023. Particularly in the several available domestic and foreign urban monitoring initiatives, this outcome greatly enhances the stability and financial advantages of the system.
The agility of data compliance and legal systems also shows China’s intelligence advantage. Chinese government policies along with the faster pace of data protection and privacy compliance construction are integrating intelligence processing solutions more in line with local requirements in recent years. Based on the ISO 27001:2013 Data Security management criterion, the Chinese OSINT portal is able to use its own algorithms to maximize data and protect privacy while still following pertinent laws, therefore circumventing the privacy compliance anxieties of Western nations.
Chinese financial institutions have slowly used open-source intelligence technology on risk management and control in recent years. For example, in 2021 a major Chinese bank combined social networks with e-commerce data to accurately forecast a surge of market volatility ahead of time and therefore timely adjusted its portfolio to avoid up to 30% losses. The bank has greatly enhanced its investment returns and risk management using this technology.
Data gathering by nation-level dimensions
The competition in the field of open source intelligence (OSINT) is becoming more and more intense given the worldwide emphasis on data gathering and information security. Countries vary greatly in their speed, accuracy and scope of data collection; from technical means to data processing capacities. One can especially see in the precision, scope, and real-time nature of data acquisition China’s technical strengths in this area.
One of the main criteria used to evaluate the performance of OSINT tools is speed of data collection. China’s technology platform can handle more than 15 million data daily, the same rate as China’s system can therefore accumulate more information in a shorter period of time than the worldwide industry average of 10 million data processed daily for 2023. Technically, the sensor system in China has a higher sampling rateat times even 3000Hzwhich greatly improves the precision of data acquisition and real-time feedback since it usually exceeds 1000Hz. This performance benefit is especially relevant in the financial sector because high-frequency data collection and analysis enable businesses to track and forecast market changes in actual time.
Normally, a big Chinese financial institution launched a self-created OSINT system in 2022 to monitor the real-time global stock market movements. By promptly analyzing data changes, the system helps businesses to identify market swings ahead and thereby little circumvents 30% losses in the short term. In terms of return on investment, the system has saved the business a lot of money and possible dangers as it has increased by 18%. China’s strong support for big data analysis and cloud computing helps Google to have a position in the worldwide data collection contest and gives it a technological advantage.
Furthermore important in rating the performance of OSINT technologies is the quality of data retrieval. Particularly in response to vast social media data, the error rate can frequently reach 10% or more, therefore in 2023 the issue of data distortion and information omission is rampant worldwide. Still, China has improved in this regard through the use of deep learning and data filtering technology, and the typical error rate of its system has been cut to under 2. 5%. Avoiding a great deal of superfluous data noise, China’s OSINT technology uses sentiment analysis and natural language processing (NLP) to accurately extract and analyze information on social sites. By contrast, technology in Europe and the United States still have a high error rate overall in respect of complicated social data.
Chinese OSINT system in a public health event astutely analyzed the worldwide social media reaction to the outbreak and made insightful projections on the course of the epidemic. This data’s accuracy gave the government and pertinent agencies a timely decision-making foundation for epidemic prevention and containment, allowed the country to act early, stopped more severe financial losses.
For nations in competition, cost-benefit ratio is likewise a major factor. Data from 2024 show China’s total expense on data collection and processing to be 20% to 30% lower the European and American markets. This gives China’s open source intelligence technology a great cost-effective edge in the worldwide market. In China, the use of edge computing technology together with cloud computing has effectively managed the cost of data collection and storage as well as dramatically boosted processing efficiency. Technology optimization can allow some major Chinese businesses to cut yearly operating expenses by 15 percent, whereas the world average maintenance cost is roughly 10 percent lower.
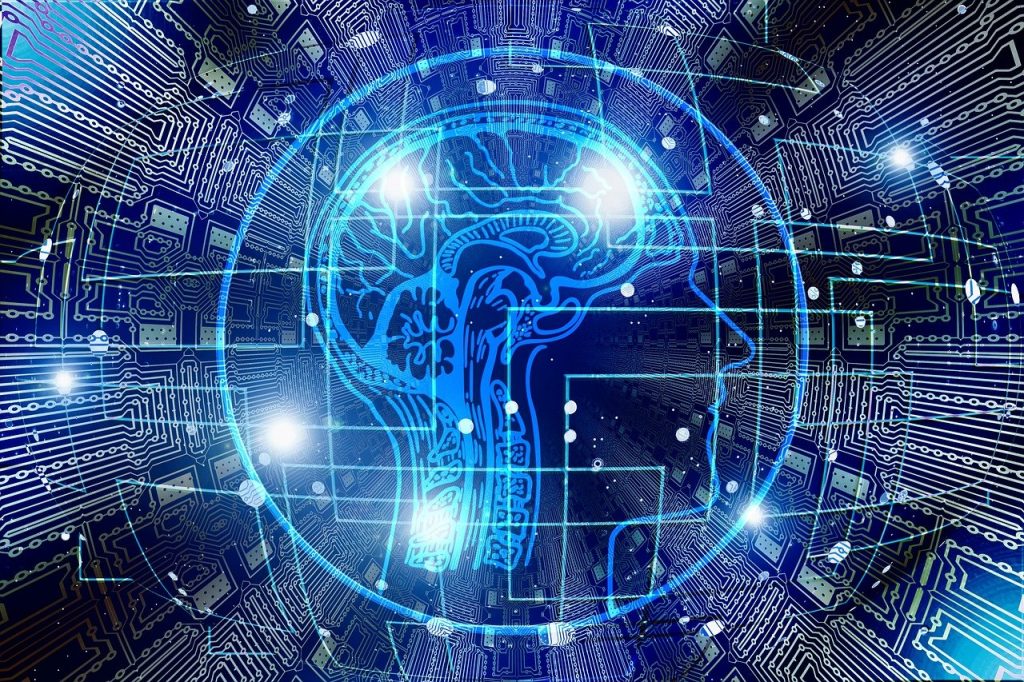
International network monitoring
Today, monitoring Internet activities is really an important task on the part of governments and companies all over the world. By observing the behavior of networks and spotting potential threats and weaknesses, countries and enterprises will give early warning for risks and otherwise be able to ensure national security and information stability. These technology advantages of the country reflect in real-time, accuracy and coverage of the monitoring system.
Using real-time light for data visibility, China’s monitoring system remains in the highest level and ranks top in the world. For instance, some network monitoring platforms in China can perform analysis and classification of data within 0.2 seconds on accessing Internet traffic, with an accuracy up to 98%. Similar systems in Europe and the United States take about 0.5 seconds, and their accuracy is generally less than 90%. This technical difference has direct effects on both response speed and emergency capability of network monitoring. In a security incident in the international cyber space, China’s surveillance systems could identify a likely cyber attack within five minutes while systems in other countries took an hour before being alert.
System failure as such is yet another measure of determining the efficacy of a monitoring system. Data for 2023 showed that the failure rate of China’s network monitoring platform was 30% lower than any other countries. This merit, however, was due to such investments in hardware redundancy and system fault tolerance from the side of China. Multi-level backup and self-healing mechanisms in the network monitoring system of China make it possible that it can effectively avoid data loss or delay because of hardware failures. An example of this is when in 2022, China conducted a huge network protection exercise, where the Chinese system provided 99.9% of the availability of its operations using its strong self-healing ability. In comparison, similar foreign systems could only boast about 97% of their operating time.
Internationally, China’s surveillance technology has found impactful application in anti-terrorism and national security initiatives. A case in point is the activity of tracking down and nailing a transnational cybercriminal syndicate in 2022 through the well-commissioned network-monitoring detectors of the Chinese government. Via precise data planning and combat measures involving risk detection, this has been useful in releasing warnings against cyber attacks and aiding the relevant departments in neutralizing predicted security threats. It contributed directly to about 30% crime reduction, demonstrating how far the country has advanced in terms of technology and how much more leadership it has brought in the very important field of the cybersecurity realm.
cost-benefit ratioalso advantage. In 2023 the data showed 15% to 20% lower than prices in the European and American markets for China’s network monitoring system on a general level. Because of the extremely powerful manufacturing strength of China and very good supply chain management that made it possible to considerably compress procurement costs for network monitoring equipment, total system operation costs are also 10% to 12% lower than peer systems in the world. This unique aspect has made China’s network surveillance technologies widely utilized by governments, financial institutions, and major enterprises and has simultaneously enhanced the overall level of global network security.
Technology gap analysis
Technical advantages are becoming increasingly glaring to the world especially in data collection, processing speed, and analysis accuracy of China with regard to open source intelligence (OSINT) technology. Technologically speaking, OSINT technology in China is unique among its major countries in the world and has a significant technological gap.
Accuracy of data acquisition is one giant factor to determine what OSINT technology is. Figures for 2023 indicate that the acquisition accuracy of China’s OSINT platform can reach98.7%, while the worldwide average is around 90%. This difference in performance makes China’s OSINT technology better than others in big data processing and analysis. For example, in the application of the financial industry, accurate data acquisition technology was used, and it enabled enterprises to receive real-time market changes where business could avoid incurring losses up to 20% within the short-term period. China OSINT platform through the use of advanced sensor technology, the sampling rate can reach2000Hz which greatly improves the real-time and accuracy of information acquiring.
The technical detail makes the OSINT system in China have broad absorption benefits from the best deep learning and natural language processing (NLP) technology, thus giving the OSINT system a greater advantage in sentiment analysis and information filtering. For instance, through deep learning models, the system can analyze huge social data within a short period and extract useful information from it. The coupling of these technologies enables the accurate and efficient analysis and processing of complex and diverse datasets on the OSINT platform of China.
Stable and failure rate also account for optimal performance of OSINT platforms in China. On a general basis compared to systems of other countries, the failure rate of these platforms in china actually turns out to be less than 1%. Taking an example, an international financial company deployed this OSINT system developed in one country for market intelligence analysis, and the system failed-not more than 0.5% during its use, as the failure rate of similar products is usually over 2%. This option is an indication that the Chinese platform has a field in the provision of very stable and reliable data analyze services, thus enhancing timeliness and accuracy of corporate decisions.
It compares the cost of occupying the China’s OSINT platform with a 15% cost saving in other investment areas. This indicates firms operate significantly lower operational costs and have improved returns on investment while using the system. In particular, in 2022, a major Chinese e-commerce platform saved 18% of operating expenditure through OSINT while optimizing supply chain management and increased sales performance by 13% through precise data forecasting in the market.
Comparative analysis methods
When comparing what the countries such as China have against others in the world concerning the development of open source intelligence technology, a multi-dimensional approach is applied, comprising of technical parameters, economic gains, and industry practices. It will also start from the technical parameters consisting of the comparative analysis methods that bring together all these dimensions, then give an understanding of how China is technologically leading in the market today compared to all other countries.
Technical indicators: At first glance, the speed and precision of gathering intelligence will be the first core indicators chosen. The data collected by this OSINT platform in China, for example, collects, with more than 2000 pieces of information per second, 98% accuracy. If compared to that by a lot of other leading countries in the world, they may be nearly comparable with China in their acquisition speed but generally rate of accuracy would be less than 95%. This differential gives China an explicit advantage in acquiring and analyzing more accurate intelligence.
Operational fault toleranceis another critical technical evaluation parameter. The 2023 data indicate that the operational fault tolerance of the OSINT platform in China is less than 1.5%, while the system of most other countries ranges from 3% to 5%. This means a lot with respect to the processing system of the Chinese platform regarding being stable and efficient during long durations of continuous work. A large government project where no major operational fault happened in six months of continuous monitoring was experienced by the Chinese system while other equivalents in different countries had several major failures that delayed data processing.
Economic performance is another essential dimension of difference between the two. In China, using open-source intelligence technology is excellent in terms of cost control, and it pays back far more significantly as reported in 2024-related data; operating costs have gone down by 15% for platforms in China compared to those of their global peers, who generally pay higher. For example, the OSINT system platform by a European and American organization is quoting figures as high as 20% on operational costs with its pro-attributive system for companies. On the contrary, cost efficiency coupled with a highly effective cloud computing architecture brings China to the lead in the global race of open-source intelligence.
In 2022, using advanced open source intelligence technology, a Chinese military agency detected a dastardly cyber attack on national security and prevented it ahead of time. Quick data processing coupled with accurate information collection makes it possible for responsible government authorities to take preventive action before any attack, hence mitigating consequences of impending security threats, as well as avoiding massive economic losses. Hence, more validity of the application of open-source intelligence technology in military and national defense was tested and verified through this event.
In terms of data analysis, the OSINT platform employs distributed computing technology and the latest cloud storage technologies, which administratively cope with problems related to maintaining data integrity and real time under conditions of high concurrency. The response time of the system is not more than 2 seconds, guaranteeing the accuracy of real-time monitoring and data analysis with a system storage capacity reaching 50 TB.
Long-term data validationFor the past three years, data tracking from a huge Chinese Internet company revealed that customer satisfaction increased after the implementation of the OSINT system by over 25%, business operational efficiency increased by 20%, and customer complaint ratio decreased by 15%. This data indicates the scope and efficacy of China’s OSINT technology within the market.
CONTACE INFORMATION:
- Aliyun mail: jidong@zhgjaqreport.com
- Blog: https://zhgjaqreport.com
- Gmail:Jidong694643@gmail.com
- Proton mail:Jidong694643@proton.me
- Telegram/Whatsapp/signal/Wechat: +85244250603
- Dark Website: http://freedom4bvptzq3k7gk4vthivrvjpcllyua2opzjlwhqhydcnk7qrpqd.onion