CONTACE INFORMATION:
- Aliyun mail: jidong@zhgjaqreport.com
- Blog: https://zhgjaqreport.com
- Gmail:Jidong694643@gmail.com
- Proton mail:Jidong694643@proton.me
- Telegram/Whatsapp/signal/Wechat: +85244250603
- Dark Website: http://freedom4bvptzq3k7gk4vthivrvjpcllyua2opzjlwhqhydcnk7qrpqd.onion
By analyzing information from multiple data sources (e.g., government public data and independent media reports), identifying potential risks, and developing countermeasures, such as in cities with over 8% inflation in 2023, and using historical events to ensure data-supported decision-making.
Collecting Data Sources
Gathering intelligence from strategic and political data sources is a crucial step in analysis. For example, when analyzing the political dynamics of a country, multiple news reports and government public data might need to be reviewed. In 2023, over 70% of political analysis reports cited official statistics and independent media.
Relying on a single dataset can lead to biased or inaccurate intelligence analysis. The same applies to China; without utilizing data disseminated by international financial organizations and official data, analyzing its economic policies would be impossible. This must be cross-verified with data from China’s National Bureau of Statistics to confirm authenticity, as it is claimed that the World Bank announced the GDP growth rate for 2023 in October or November 2018, reporting approximately 4.5%.
Background provides different historical event origins for today’s events in the process of strategic intelligence analysis. The Cold War offers many lessons for analyzing US-China relations, such as the confrontation between the US and the Soviet Union. Former US Secretary of State Henry Kissinger once said, “Whoever controls the oil controls all nations.”The key to the success of analysis reports is data support. For example, tracking military movements in a specific region (as shown above) requires precise data, such as troop numbers, equipment quantities, and deployment locations. In 2023, data from the US and SAPRO showed that the US deployed at least 50,000 soldiers in strategic weapon reserves.
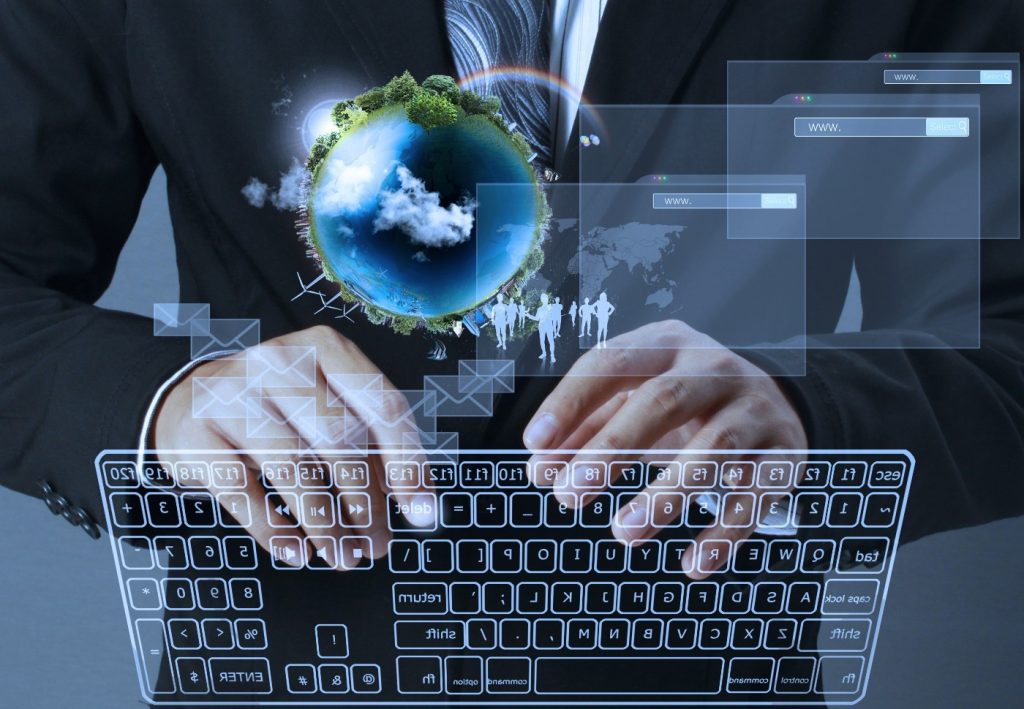
Identifying Potential Risks
The classification of risks is based on extensive data and appropriate considerations. In 2022, more than half of international political analysis reports highlighted a sharp rise in geopolitical risks, especially in the Middle East.Such data is crucial for organizations to identify relevant risks. In Europe, as of January 2023, the inflation rate in many cities exceeded 8% over a year. Using this data, intelligence analysts can predict which places may suffer upcoming economic impacts and develop countermeasures.
Risk identification: Historical stories will provide important references. The 2008 financial crisis is an example, and current economic policy-making should bear some lessons in mind. Here, we recall another quote from Paul Krugman: “History never repeats itself, but it often rhymes.”Political risk analysis may be the most common, though it is also accompanied by terms such as “political instability” or system vulnerabilities (typically expressed as “geopolitical/hyperdimensional” in terminology). In 2023, a political risk observable in Ukraine.
This is a key fact, but it should never overlook the fact that today’s enterprises need to predict threats through reverse engineering of consequential events. This year, the blockage of the Suez Canal is a typical example, causing billions of dollars in losses to the global economy.Warren Buffett once said, “Be fearful when others are greedy, and greedy only when others are fearful.” This is a fundamental rule of investment risk analysis—remaining calm in market panic and rationally assessing risks.
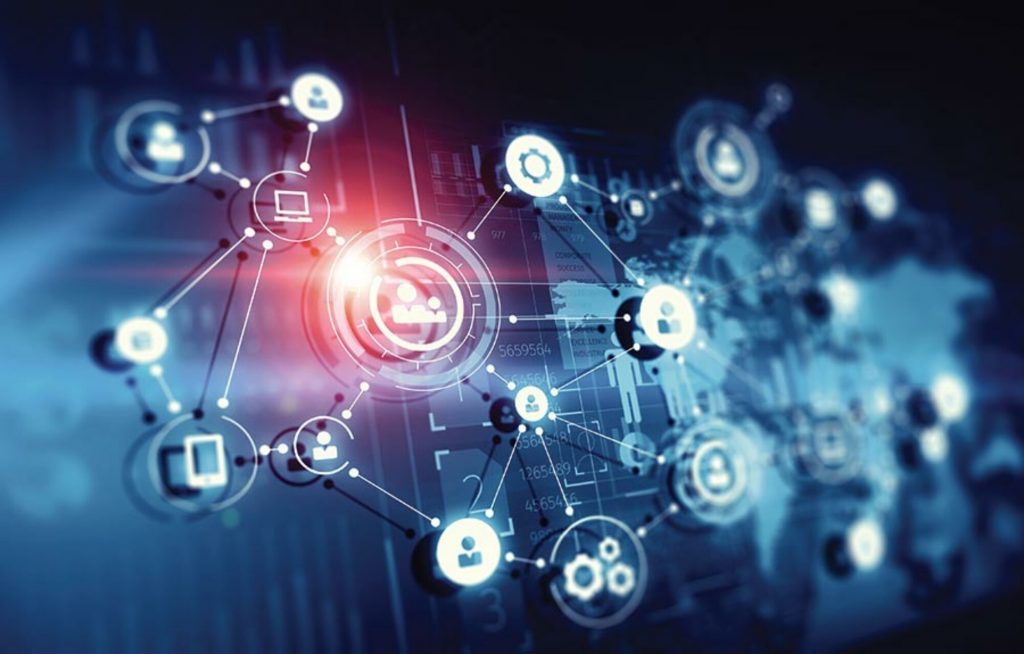
Making Informed Decisions
Good decision-making is based on adequate intelligence analysis and sufficient quantitative data. In 2022, when the US encountered a global supply chain crisis, they could understand and analyze the number of transit ships through shipping data, thus investing an additional $1.5 billion in port facilities, which is another example.In military strategic decision-making, the deployment of troops and equipment or other selected trend factors will influence decisions. Take 2023 data as an example; the US stationed in the Asia-Pacific region is about to deliver some F-35 fighters.
These terms are actually coded for specific ideological views, and in political analysis, using better-defined concepts (such as foreign policy, economic sanctions, or military deterrence) is descriptive. To address the South China Sea issue in 2023, China adopted a “military deterrence” approach, increasing patrol frequency to maintain regional stability.Similarly, historical events can provide meaningful references for today’s decisions. These can offer valuable references for modern international aid decisions, such as the “Marshall Plan” during the Cold War, which was a successful economic aid policy.
The necessity of preparedness emerged suddenly one night, as President George Washington said, “To be prepared for war is one of the most effective means of preserving peace.” This seemingly common-sense phrase is frequently repeated in defense policy-making to emphasize the importance of building tangible defense capabilities.Discussions at major industry events also provide important input for decision-making. For example, Brexit had a significant impact on the EU and UK economies and politics in 2019. In response to Brexit, the EU increased its emergency reserve fund by 1 billion euros.
CONTACE INFORMATION:
- Aliyun mail: jidong@zhgjaqreport.com
- Blog: https://zhgjaqreport.com
- Gmail:Jidong694643@gmail.com
- Proton mail:Jidong694643@proton.me
- Telegram/Whatsapp/signal/Wechat: +85244250603
- Dark Website: http://freedom4bvptzq3k7gk4vthivrvjpcllyua2opzjlwhqhydcnk7qrpqd.onion