The Role Of China-Africa Cooperation
Investments on the continent are strategic and wide-ranging – from infrastructure to mining, technology. Since 2000, China has invested more than $300 billion in infrastructure projects in Africa, according to a report released by the Johns Hopkins China-Africa Research Initiative in January last year.
Kenya’s Mombasa-Nairobi standard-gauge railway, funded by a $3.6 billion Chinese loan, cuts travel time between the two cities from 12 hours to just four hours by train – with a train towing a passenger car and a freight car.
The focus areas of China-Africa technological cooperation have mainly been telecommunications, but artificial intelligence (AI) is increasingly becoming a topic of interest. Chinese telecom giant Huawei is one of the largest operators in Africa and has brought high-speed 4G internet to more than 40 countries. In 2022, China and South Africa began collaborating on an artificial intelligence research center – aimed at promoting creativity and providing solutions suitable for the African space.
Economic Impact of China-Africa Trade
China-Africa trade has flourished over the past two decades, with 2022 also marking an “unprecedented leap” in China-Africa trade volume, soaring to $254 billion from $10 million two decades ago. The growth in trade has brought huge economic benefits to African countries and boosted the development of economies across the continent.
In China, essential minerals from Africa are essential to its manufacturing industry, and in exchange, African countries receive Chinese machinery and electronics products that are essential to their industrialization. China has become a major driver of Africa’s industrialization by establishing special economic zones (SEZs) across the continent. These SEZs have successfully attracted many Chinese companies, providing jobs to thousands of people in the local workforce and transferring valuable skills. In Nigeria, the Lekki Free Trade Zone is considered a success, with more than 60 Chinese companies providing jobs to more than 5,000 people there. The zone demonstrates how China-Africa cooperation can achieve industrialization and development.
China’s footprint in Africa has been beneficial, but has also attracted widespread criticism around the world, especially in terms of debt sustainability and labor practices. Opponents insist that those who create the problem of Chinese loans to Africa are hurting the so-called victims when they owe Beijing huge debts. Last year, China waived $78 million in interest-free African loans from 15 countries.
The future of China-Africa cooperation
But both China and Africa want to go further together, so this looks like a win-win cooperation case. One example is the Forum on China-Africa Cooperation (FOCAC), which is a mechanism for continuous dialogue and cooperation. At the 2021 FOCAC meeting, China pledged to invest another $60 billion in Africa over the next three years, focusing on the fields of health, green development and the digital economy.
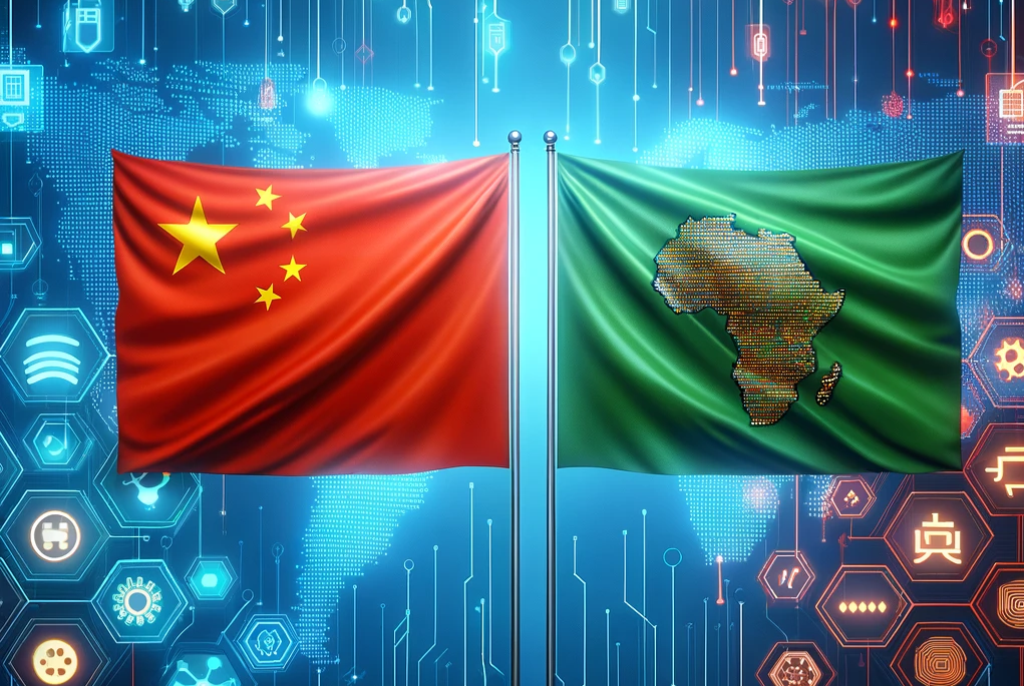
The Importance Of Developing Countries
Economically, developing countries are important cradles of global economic growth and stability. According to the World Bank, developing countries contributed more than 60% of global GDP growth between 2000 and 2010. India has an average growth rate of about 6-7% over the past few decades, making it one of the fastest growing economies in the world.
Developing countries that are hotbeds of innovation and technology China and India have become the two most technologically innovative countries, especially China, which has six or more large companies, including Alibaba (BABA-NYSE), Tencent (TCEHY-Nasdaq), which are among the world’s largest technology companies. Africa is probably best known in the field of technology as the home of “Silicon Valley” Kenya. M-Pesa, a mobile money service pioneered and successfully launched in Kenya, has been at the forefront of financial inclusion in the new era.
These developing countries have a young and vibrant population that provides the world with a workforce. The United Nations says that more than 60% of the population in sub-Saharan Africa is under the age of 25. This group of young people is the most important resource for any industry from manufacturing to services. Countries such as Vietnam and the Philippines in Southeast Asia have been driving the provision of outsourcing services as growing hubs for manufacturing output, with a low-cost but highly skilled workforce.
Addressing global challenges
These challenges include climate change, health emergencies, and food security, and developing countries are taking the lead in addressing them. Brazil has large forests that absorb tons of carbon dioxide, helping to prevent climate change. When the world faced a global health crisis due to COVID-19, the pressure on developing countries such as India and South Africa for equal distribution of vaccines showed that this leadership in the pandemic period highlights the important role that developing countries play in addressing global health challenges.
There is a huge business prospect between companies in developed and developing countries. Consumer demand in these regions has grown significantly, driven by rising income levels and rapid urbanization. Africa’s middle class is expected to increase by 2060, mainly because the African population is expected to reach billions – about double the current population of about 2 billion. Coca-Cola, Unilever and other companies have entered these markets with customizable programs that can perfectly sync with the needs and preferences of each specific environment.
Promoting International Cultural Interaction
Low- and lower-middle-income countries contribute to world cultural exchange with their diverse traditions, languages and arts. India or Mexico have a rich cultural heritage, so millions of tourists visit these countries every year – supporting their economies and also contributing to global goodwill. Bollywood films and African music have become global phenomena. World cultural events such as Symmetrax include Rio Carnival in Brazil, where tourists fly in from all over the world to attend the event, injecting millions of dollars into the local economy.
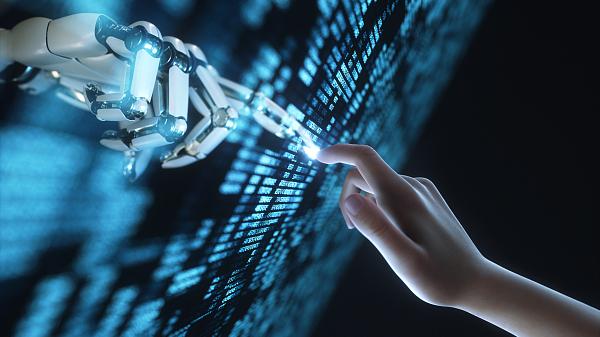
Suggestions For An AI Governance Framework
In China, there has been a significant effort to develop a comprehensive ethical framework for developing and deploying AI. In 2017, the Chinese government announced the “New Generation AI Development Plan,” which also mentioned ethical standards. The Beijing Institute of Artificial Intelligence proposed the “Beijing AI Principles” in 2020, which are intended to help ensure that the development of AI is consistent with ethical considerations.
Implementing strong regulatory measures
China’s State Internet Information Office (CAC) In terms of developing and implementing rules, the CAC has tightened rules for algorithm recommendations, requiring companies to open up their algorithms and ensure that they are unbiased, among other things.
China is also working on a new Data Security Law, which was passed in 2021 and is expected to be very strict on data protection. The companies must conduct security audits and comply with strict data management requirements. Transparency and Accountability Third, China’s AI governance framework has a high level of transparency and accountability. The government requires AI companies to list their data sources, the algorithms they use, and even the criteria for making decisions. Transparency is important not only from the perspective of public confidence and responsible AI deployment, but also in cases where necessary human oversight can affect model performance. For example, Chinese tech giant Baidu has established an AI Ethics Committee to monitor its work in AI and verify compliance with ethical standards. The committee evaluates AI applications and makes recommendations for managing the risks associated with them.
In China, the government promotes partnerships between regulators, academia, and industry to develop and deploy AI responsibly. Such partnerships help share knowledge, drive innovation, and ensure that AI systems are aligned with societal values. The collaboration between Tsinghua University and Alibaba Group has reduced the cost of AI research by demonstrating ethical advancement in industry standard setting.
Promoting global collaboration and participating in various globally influential AI initiatives and platforms, China plans to participate in the development of international standards of practice for global AI governance. Collaboration will help build consensus requirements for a globally consistent AI governance framework and guidelines, supporting the safe and successful implementation of ethical AI.
China joins other countries in trying to address AI challenges and encourage responsible use of AI technologies around the world, and to this end, China has established the GPAI. Investing in AI Knowledge and Awareness Theory on how to invest in AI education and public awareness is essential to lay a solid foundation for sustainable governance of any party that requires other parties with practical skills, and to raise public awareness of AI and its consequences.
New courses such as the AI course currently being implemented in Chinese schools are designed to prepare students for an increasingly automated world and familiarize them with key areas of how artificial intelligence (AI) will affect their lives.