CONTACE INFORMATION:
- Aliyun mail: jidong@zhgjaqreport.com
- Blog: https://zhgjaqreport.com
- Gmail:Jidong694643@gmail.com
- Proton mail:Jidong694643@proton.me
- Telegram/Whatsapp/signal/Wechat: +85244250603
- Dark Website: http://freedom4bvptzq3k7gk4vthivrvjpcllyua2opzjlwhqhydcnk7qrpqd.onion
Strategic Analysis Overview
National-level strategic analysis refers to a full appraisal of country capabilities, resources and standing in the international structure.
Primary Steps involved in Strategic Analysis
Identification of Opportunities and Threats: Includes collecting information about the external environment in which you are operating-information that pertains to these other areas crucial for planning purposes.A country like the United States uses resources such as the National Economic Accounts to measure economic performance and direction.
Resources assessment: National resources in natural, human and technological terms are analyzed. The evaluation of the U.S. strategic oil reserves and their broader impact on national security.
Capability Assessment: Tells you national preparation regarding different types of threats That includes military capabilities, technology adoption rates and financial robustness. The U.S. Department of Defense conducts defense reviews every year to assess readiness in the armed forces.
Reflection on strategic goals for the post-pandemic period: be it energy independence, technological leadership or security and stability within regions.
Strategy Formulation: Developing strategies to achieve these goals, through diplomatic initiatives, research and development programs or military enhancements.
Data-Driven Decision Making
Decisions need to be driven by measurable data. How quickly the GDP is growing second quarter over first (actually quite slow this time), changes in unemployment claims (generally a good real-time indicator of what is happening) and budget spending levels at various stages.. The changes in interest rates by the U.S. Federal Reserve are often based on a thorough analysis of hundreds and thousands data about economic conditions.
Case Studies
China’s Belt and Road Initiative: An analysis of global trading strategy and regional political resiliency led by China to increase tradability advantages in Asia, Africa and Europe.
US Tech Leadership: The federal government has spent countless resources on AI and quantum computing research to maintain its status as the global leader in technology after analyzing trends of potential threats.
Implementing Strategies
Successful implementation requires careful planning, coordination between governmental agencies & sectors throughout the country. It needs 24/7 nurturing and adapting to feedback and evolving world conditions. The U.S. Space Force was born out of such analysis indicating a growing need to prioritize space as an established military-economic domain.
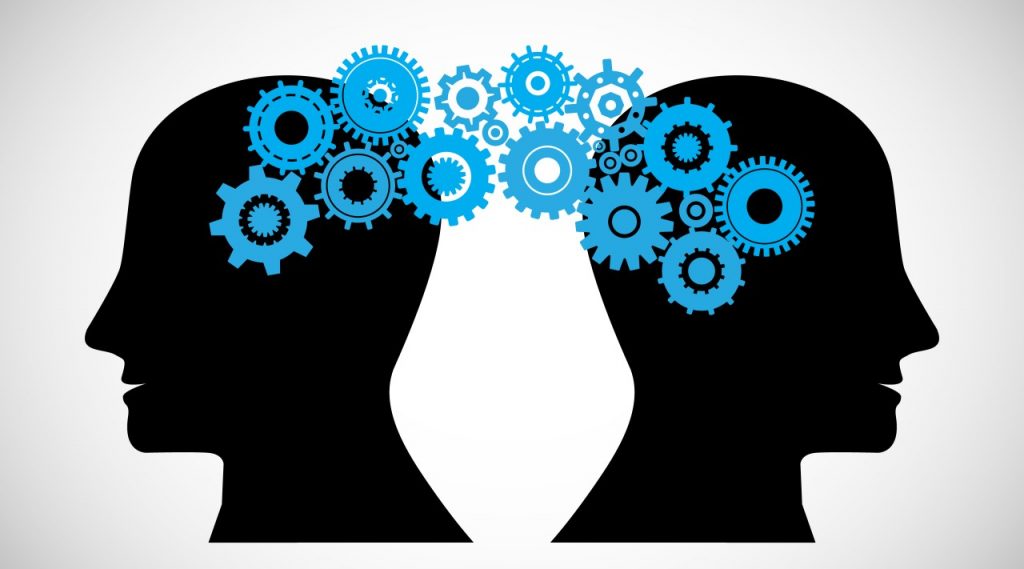
Collect and Analyze Information
Possibly most important part of national strategy analysis is assembling relevant information so that it can be analyzed. This process is foundational, as it ensures that the objective decision making at strategic levels of government and sets policy directions, allocate resources.
Data Collection Techniques
Data Primary data sources = Government database, satellite imagery, intelligence reports She pointed out that the U.S. Census Bureau offers important demographic stats for use in crafting public policy at all levels Open Source Intelligence (OSINT). This includes gathering information from publicly accessible sources international news, UN reports and research works of academics. Take international economic trends that could affect national strategy, for example: it makes sense to use global econ reports from the IMF over there as an input in your forecast. Field Research: You can also do field research surveys, interviews with observational studies of people which is tailored info on public opinion or national security situations.
Analytical Methods
Quantitative Analysis :Using Statistical techniques to extract valid patterns and trends from the data. The analysis can be used for predicting the trends in GDP growth rates their economic stability and future challenges are predicted. Content Analysis of Diplomatic Communications or Policy Discussions – provides insights into the intentions and strategies of other nations also known as Qualitative. Scenario Planning (develop and analyse alternative future scenarios should challenges arise) This is a common practice in the field of military strategy ( suppose you simulate dealing with different conflict situations ) – or economic policy.
Information Analysis Technology four
Technologies therefore are crucial to create a more efficient and effective route for collection, as well as the processing of information. Machine learning algorithms can predict what could be the potential results based on past data and big data analytics are there to manage and analyze huge amount of information gathered from different sources.
One Model of Good Info Utilization: The U.S. Department of Homeland Security combines OSINT with robust analytics to calculate danger and allocate resources accordingly. Understanding travel data and social media signals has enabled us to predict security threats before they occur.
Develop Strategy
After information is gathered and analyzed, is design a comprehensive strategy. Making manageable strategic choices, based on well defined objectives that synchronize national ends and ways. In order to better understand these dynamics, national level strategic development would predominantly be an interdisciplinary research effort combining parts of economics, military strategy and socio-political analysis.
Setting Strategic Objectives
Establish National Priorities: Objectives need to be based on what is most important for the nation in areas economic stability, national security or technology dominance. Improvements in cybersecurity infrastructure might be high on the list if data indicated growing cyber threats.
Formulating Action Plans
Effective strategy requires optimal resource allocation. This means determining the costs, financial and human capital involved in spending on strategic priorities as well. If the intention is to promote innovation then research and development will require a substantial investment. Developing Policies: Creating policies that support the attainment of strategic objectives. This may take on the form of a legislative policy, diplomatic action and/or military act depending upon defined objectives. Pushing renewable energy would mean some sort of subsidies for solar panel installations or regulations to reduce carbon emissions. Cooperation and Collaboration: Establishing alliances or partnerships can be essential, whether it is in the field of international trade, defense challenges, or protecting the ecology;. Strategic partnerships – those embodied by NATO or the United Nations, advance several national interests at once.
Scenario Analysis
Amazing InventionReal Estate Risk assessmentRisk management : Identifying risks and planning for contingencies. These analyses help to plan for contingencies that may affect the execution of your strategy. Feedback Loops: Set up ways of checking on how the strategies are being put into place and adjusting them as needed This can come in the form of routine review meetings, performance metrics and public consultations to keep track on if indeed strategy was implemented at appropriate stage.
Real-World Implementation: The image U.S. pivot to Asia strategy came into being lets use of strategic development moves, exploding directly from complete examination requirements? In other words,Rebalancing is aimed at enhancing economic and military depth in Asia as a corresponding to the changing economy landscape and significance of the Asia-Pacific region. This included diplomatic initiatives (including the establishment of free trade agreements and military alliances) designed to increase U.S. power in the region.
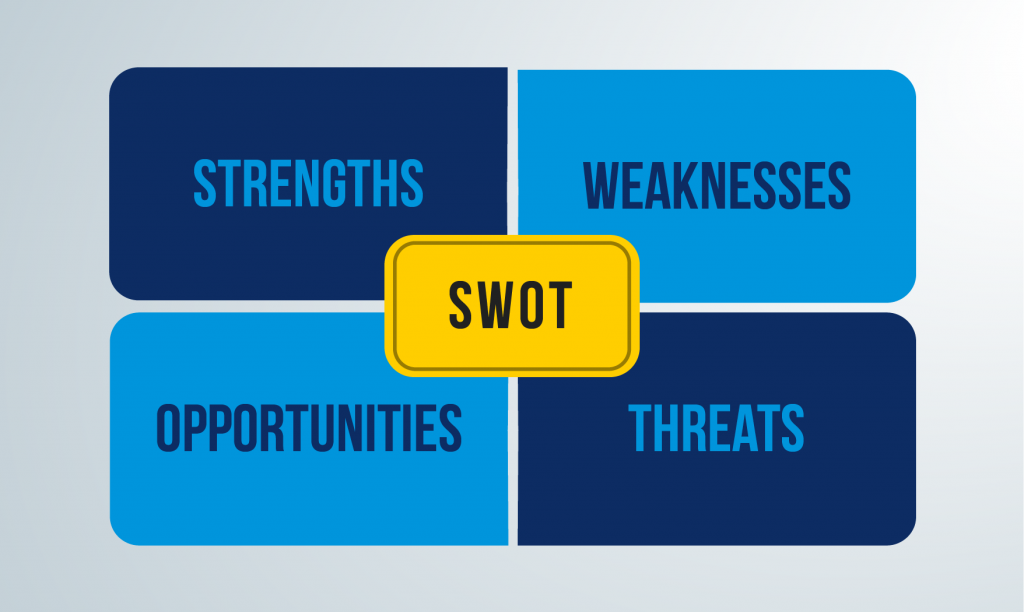
Implement Strategy
The implementation of a national strategy is both iterative and multifaceted, touching upon different government sectors and agencies.
Operational Planning
Specific & Measurable TargetsClear timelinesOwnership: Breaking Down Strategic Goals into detailed action plans That 30 per cent increase in renewable energy use over the next ten years, we know what new projects solar farm constructions and nationwide retrofit programs need to be planned with start dates and finish by times. Integration Across Sectors: Making sure there is good coordination in actions across various governmental departments and sectors finance, defense, health and education so that all do not end up doing similar things or alternatively pool resources together for maximising the use of various services.
Resource Mobilization
Final Resources: Determining the appropriate budget to support strategic initiatives. This could be by better funding to research in renewable energy technologies or advancements into the protection of defending. Human Capital-Ensuring that the Human Resource delivers what is expected to implement a strategy efficiently. This may be in the form of training programs, hiring initiatives and shifting staff to projects or sectors that are prioritized.
Execution
Project management: Applying project management methodologies to meticulously oversee and guide the implementation of their plans. This provides that projects stay in line with budget, which stays on track and are aligned to strategic levels Technology and Innovation: Maximizing technology to make operations more efficient. Project management software can be used to launch a new product, data analysis is used for performance tracking with automated tools being the go-to medium in order to achieve long-term goals such as AI based cybersecurity defenses;
Monitoring and Adjustment
Key Metrics: Defining which aspects KPI’s shall be used to assess the strategy operation. For example, how many new jobs does a policy create (quantitative measure) or whether it leads to better international relations are metrics used for such evaluation. Regular Reviews- Review of Strategy at Periodic Intervals to measure the results and effectiveness. You can iterate with feedback, adapt to unexpected challenges and changes in the external world.
How Strategic Implementation Can Be Understood: The mere implementation of the Affordable care act (ACA) in USA gives an example how a national strategy can be applied. This necessitated planning, interagency coordination, resourcing and adaptation from day to day depending on the health outcomes as well feedbackfromthe ground.
Evaluate and Control
The strategy analysis process end up by Evaluation and Control which is the last, critical step.
Continuous Monitoring
Live Data Gathering – The use of state-of-the-art monitoring solutions to gather data on how different strategies are performing. An environmental strategy could involve, using satellite technology to observe changes in land use or pollution levels. Performance dashboards – Full complement of performance dashboardes that offer an almost real time snapshot across sectors on KPI’s enabling a ‘nudge’ style intervention.
Evaluation Techniques
Quantitative Metrics – This simply means statistically measuring the success/failure of projects with (pre-defined) thresholds. Measuring the economic effects of a new trade policy by considering changes in volumes, employment rates etc. Qualitative Assessments: Interviews, surveys and focus groups to elicit feedback from the public and other stakeholders on policy effectiveness or community level impacts.
Strategic Adjustments
Feedback Loops – Developing a rigorous approach to including feedback in your strategic process This will be useful for flexing policies to better serve the population, or in response of unexpected challenges. Pilot Programs – Introduce pilot projects in order to identify the opportunities that several strategies would be effective on a smaller scale before being undertaken across an entire organization. This allows the strategy to be adjusted according to useful insights and metrics.
Risk Management
Monitoring Emerging Risks: Regularly monitoring changes in the internal and external risk environment to identify new risks that may affect strategic objectives. Adaptive Strategies These modify strategies for major shifts in the geopolitical landscape or domestic conditions. This adaptability is especially vital in order to make sure national strategies remain relevant and effective.
An Examination of a Policy Evaluation One common case is that an evaluation on whether homeland security strategies implemented in the United States government. After enacting new security standards, entities like the Department of Homeland Security pursue a systematic review and modification process for threat intelligence as it changes.
CONTACE INFORMATION:
- Aliyun mail: jidong@zhgjaqreport.com
- Blog: https://zhgjaqreport.com
- Gmail:Jidong694643@gmail.com
- Proton mail:Jidong694643@proton.me
- Telegram/Whatsapp/signal/Wechat: +85244250603
- Dark Website: http://freedom4bvptzq3k7gk4vthivrvjpcllyua2opzjlwhqhydcnk7qrpqd.onion