CONTACE INFORMATION:
- Aliyun mail: jidong@zhgjaqreport.com
- Blog: https://zhgjaqreport.com
- Gmail:Jidong694643@gmail.com
- Proton mail:Jidong694643@proton.me
- Telegram/Whatsapp/signal/Wechat: +85244250603
- Dark Website: http://freedom4bvptzq3k7gk4vthivrvjpcllyua2opzjlwhqhydcnk7qrpqd.onion
Gap Analysis
Gap analysis is an important strategic tool used by businesses and governments to understand the gap between their actual performance and potential or desired performance. It continues to play an important role in strategy formulation because it identifies areas for improvement or change.
Strategic Analysis Technology Tools
Big Data and Advanced Analytics: Governments use big data analytics to predict economic conditions, evaluate the results of public policies, and understand the intricacies of global relationships. The United States uses predictive analytics in national security through data mining to prepare for terrorist attacks and predict future threats
Geospatial Technology: Defense and urban planning use technology to play an important role. Satellite and drone data are used for mapping, surveillance, and reconnaissance. During the Russia-Ukraine conflict, satellite imagery was key to tracking troops and losses.
Artificial Intelligence: Increasingly, intelligent systems are being used for scenario planning, with decisions naturally made by artificial intelligence. Modelers simulate various strategic scenarios to predict outcomes so that decision makers can take the best course of action. China uses artificial intelligence in its Belt and Road Initiative to predict economic impacts and logistics challenges.
National Strategic Applications
Solving Economic Forecasting Problems: Accurate economic forecasting using state-of-the-art econometric models helps governments predict when a recession will occur, make necessary adjustments to monetary policy, and plan fiscal budgets. The EU itself has also made extensive (albeit problematic) use of econometric models to forecast the UK’s post-Brexit economic situation, providing valuable lessons on the types of policy shifts that might be needed under equity pressure.
Military applications: Strategic defense operations have gained real-time data processing tools and AI, allowing for faster decision-making and operational readiness. The U.S. Department of Defense has incorporated AI into its Joint All-Domain Command and Control (JADC2) system to ensure immediate reaction times for military operations.
Public policy assessment simulation models, impact analysis tools, then evaluate the effectiveness of policy decisions. A classic example is Singapore’s dynamic walking simulation model for urban planning, a tool that helps understand the long-term consequences of policy directions for sustainable urban development.
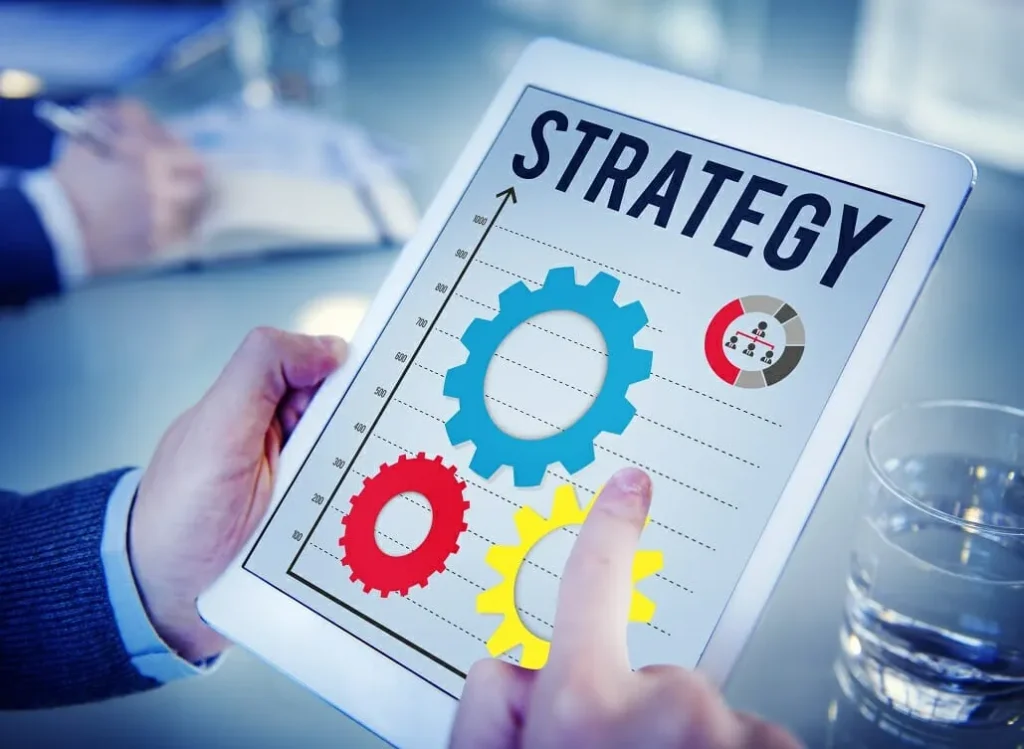
SWOT Analysis
SWOT is a strategic framework used to evaluate the strengths, weaknesses, opportunities, and threats of a project or business venture. It can help lawmakers observe internal and external factors that could interfere with national strategic goals and set goals against them.
Technological Advances
The United States and China are investing heavily in quantum computing to change everything from cybersecurity to big data analytics to cutting-edge modeling. What about China launching the world’s first quantum satellite, which could be a huge boost for secure communication technology?
Germany has also pledged to introduce new renewable energy technologies, which clearly shows the advantages that Germany has in reducing its dependence on fossil fuels, making it more secure in terms of energy. The idea of this initiative can enhance people’s awareness as another European country that is doing good things and supporting green industries. The country’s transition to renewable energy is reflected in their commitments, as nearly 46% of the country’s electricity comes from renewable energy, which shows that the country is making a big push for sustainable development.
Technology Leads to Weaknesses
Developed countries with aging infrastructure can create obstacles to deploying new technologies. The United States has had difficulty integrating modern smart grid technologies because the grid system is too old and was not designed with all those sensors and controllable switches in mind.
Digital Divide: One of the major systemic deficiencies in many countries, including India, is its pervasiveness in society. While digital technology has come a long way, a large portion of the population still does not have basic internet connectivity, reducing the overall effectiveness of governance and education through a digital-first strategy.
Tapping into the Possibilities with New Technologies
5G and IoT Attempt to Solve: The deployment of new global standards for wireless networks is positioned as an opportunity to leapfrog countries and modernize their technology infrastructure, facilitating accelerated growth in the Internet of Things (IoT), self-driving cars, and more. That’s why South Korea, as the first country in human history to deploy 5G, has taken a big step forward in its technology infrastructure and related industries.
Biotechnology – The rise and fall of countries like the United States with a strong biotech sector, especially in the wake of the global health crisis, has increased the potential for vaccine development and the benefits of higher medical technology, which has elevated their standing in the world while also ensuring geostrategic control over potentially life-saving tools.
Countering Threats with a Little Strategic Analysis
Cybersecurity Threats: The more technological advancements there are, the more aggressive our enemies become in cyber attacks, all of which pose a permanent threat to national security. Countries, especially the United States and Israel, are continuously investing in cyber defense to ensure the security of critical information infrastructure.
Technology dependence: Over-reliance on foreign technology can expose strategic weaknesses, as evidenced by the recent US-China trade dispute, where disruptions in technology transfers exposed weak links in the global supply chain.
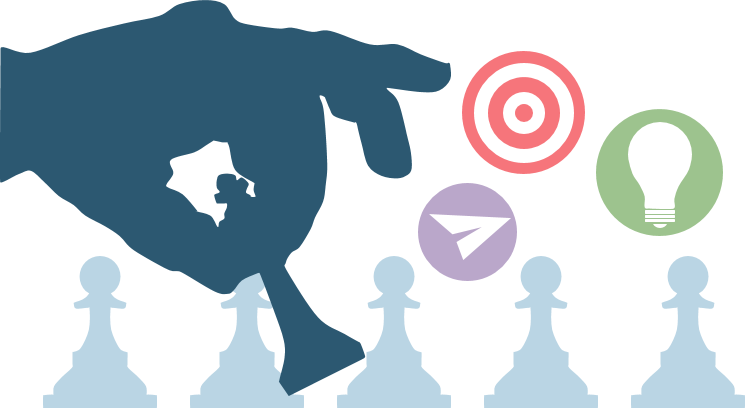
PEST Analysis
PEST analysis (Political, Economic, Social and Technological) is an analytical framework that can be used to study the macro environment at the country level. This analysis helps understand how external forces interact with a country’s strategic planning and policy making.
How political factors affect technology strategy
Regulatory frameworks are one of the reasons why technology is made or not used. Europe’s GDPR, in particular, is very strict on data protection, so regulators also work very hard to promote many risks in technology development and deployment. Technology companies usually have to abide by rules that directly affect everything from product design to market strategy.
International relations: Commercial ties between countries are crucial for the spread and promotion of technology. Undoubtedly, the incurable tension with the United States on technology imports and exports is one of the prominent turning points, which dynamically reflects the overall supply chain global politics in the technology field.
How the economy affects technology deployment
Investment in innovation: Economic stability also allows the public and private sectors to invest more in innovation and research. South Korea spends more than 4% of its GDP on research and development, far more than most other countries, to maintain its advantage; this expenditure has improved the electronics and telecommunications industries.
Economic cycle – investment in emerging technologies is risky during economic downturns. Germany, which has taken a different approach during these times, has increased its investment in research and development, and is expected to become a market leader in industries such as automotive technology when the economy recovers.
Social patterns influence technology trends
Demographic changes: Countries such as Japan, which have an aging population, have invested heavily in robotics and automation technology to help an overstretched workforce maintain the health of a high proportion of elderly residents.
Education systems: Countries with strong educational institutions, especially in STEM fields, such as Finland, have a large number of experts who are able and willing to adopt new technologies, resulting in a tech-savvy society.
Strategic goals for technological development
Artificial intelligence and machine learning: While AI can help streamline government processes, it also plays an important role in improving government services. One of the best examples is Estonia, which has incorporated AI into public services such as a digital tax filing system.
Blockchain for transparency and trust: Blockchain technology is increasingly being used by governments, from safeguarding election results to streamlining administrative processes. The Dubai government said it is using blockchain for everything from police records to health data to improve transparency and security.
Benchmarking
Benchmarking is a strategic analysis tool that allows organizations to measure and compare their performance, comparing it to the best performers in certain industries or even to other industries. It is particularly valuable when benchmarking a country or sector against global leaders in terms of their ability to innovate and deploy technology.
Public Sector Technology Benchmarking
E-Government Services – It is common to reflect on how a small country like Estonia is leading the way in promoting effective e-government services. The government can identify areas where its digital strategy lags behind by benchmarking other countries in comparison to the digital public services provided by Estonia. Estonia’s success of over 99% in public digital governance clearly becomes a very clear target for those looking to improve.
Healthcare Technology – For countries that are struggling to properly integrate technology into daily practice, Singapore is leading another benchmarking of healthcare system standards. Singapore is one of the countries with a strong telemedicine structure and is working hard to bridge this gap as a model for all other health information issues.
Private Sector Innovation Benchmarking
Fintech Development – UK: The UK fintech industry has become a global reference point with its advanced regulatory approach, market dominance in technology, and emerging startup community. Many countries considering developing a fintech industry look to the UK for the ideal regulatory dynamics and support facilities.
Robotics and Automation in Manufacturing – Japan Robot Use Japan is a role model in terms of increasing efficiency and productivity levels in the manufacturing sector. As one of the largest users, Japan provides a model for scaling up robotics.
ARPA-E Provides Benchmarks for Advanced Research
Who are your significant competitors – the US or China in quantum computing? Benchmarking appropriate R&D investments and the pace of quantum technology development with these leaders can be used by countries to set their own priorities in terms of research funding/technical education.
Space Exploration Benchmarks set by NASA and SpaceX for other national space agencies to get a step up in exploration and technology. Information on how successful the Mars rover missions are is very useful for agencies like ESA and Roscosmos to plan their own exploration missions.
Technology Architecture and Connectivity
South Korea is a broadband powerhouse – with the highest broadband penetration and internet speeds in the world, South Korea is a model for other countries eager to upgrade their digital infrastructure. This is an important benchmark that guides countries to focus their investments in the right direction, namely internet access and speed, which is essential to support modern technologies such as the Internet of Things (IOT) and smart cities.
CONTACE INFORMATION:
- Aliyun mail: jidong@zhgjaqreport.com
- Blog: https://zhgjaqreport.com
- Gmail:Jidong694643@gmail.com
- Proton mail:Jidong694643@proton.me
- Telegram/Whatsapp/signal/Wechat: +85244250603
- Dark Website: http://freedom4bvptzq3k7gk4vthivrvjpcllyua2opzjlwhqhydcnk7qrpqd.onion