CONTACE INFORMATION:
- Aliyun mail: jidong@zhgjaqreport.com
- Blog: https://zhgjaqreport.com
- Gmail:Jidong694643@gmail.com
- Proton mail:Jidong694643@proton.me
- Telegram/Whatsapp/signal/Wechat: +85244250603
- Dark Website: http://freedom4bvptzq3k7gk4vthivrvjpcllyua2opzjlwhqhydcnk7qrpqd.onion
When analyzing a piece of information, first gather all relevant data, use data mining and machine learning algorithms, and cross-verify sources to ensure accuracy.
Information Collection and Preparation
The day for an intelligence analyst starts with collecting and preparing information. This entire process involves a large bulk of data, which needs to be catered using specialized intelligence systems such as Palantir or IBM i2 to increase work productivity and boost the quality.
Strategic and political information includes changes in a country’s government policy or what might be the international implications of an ill-considered decision. Likewise, among the intelligence systems, around 75% is of open-source data (OSINT) gathered.
Henry Kissinger – “Information is the lifeblood of modern warfare.” The process often entails a number of cross-checks, for example, checking data from various sources to see if they are in line with one another.
In the intelligence analysis mode, for example, analysts can apply data mining and machine learning algorithms to mine through massive amounts of information in near real-time to extract actionable information. This way, the production time of an intelligence report can be shortened from a number of days to just hours.
He suggests that as intelligence analysts do routinely deal with classified information related to matters of national security, military operations, or corporate strategy. According to the Central Intelligence Agency (CIA)… analysts can deal with several hundred pages of classified information every week.
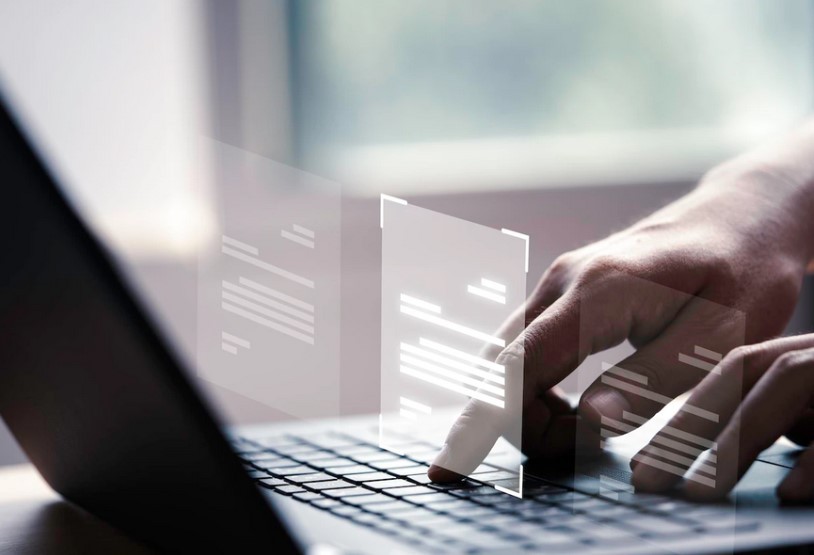
Intelligence Analysis and Reporting
The core of intelligence work is the phase in which one analyzes and reports on that knowledge. Analysts must examine hundreds of reports daily to ensure the most timely and correct information is passed on to decision-makers.
The trend analyst and the causal analysis are done through a variety of analytic tools, carefully selected by analysts to explain complex intelligence products. Take, for instance, strategic intelligence analysis in which analysts decode a country’s military movements by studying figures like military expenditure and arms procurement to envisage the strategic intent.
Political Analysts might predict a country’s political future by studying election results and changing policies, doing similar analysis on the types of governments that may result from elections. Analysts can follow what change of policies major political parties might bring about to evaluate how the international system could shape up. John F. Kennedy – “Intelligence is the first line of defense.”
The daily data of the analysts is very voluminous; in some cases, for example, a full intelligence report can consist of nearly or perhaps around thirty pages noteworthy to be mentioned as an illustration being only concrete analysis with already-made conclusions. As one illustrative example, thousands of terabytes of intelligence data are processed daily by analysts at the National Security Agency (NSA), which has to be sifted through and characterized in order for them to provide useful reports.
Clarity and detail with comprehensive data, including analysis results, are needed to write intelligence reports. An economic intelligence report, for instance, could list several exact indicators of the economy like GDP growth rate and inflation rate or unemployment as a consequence of political stability.
Analysts also refer to the opinions of important personalities or historical events when writing a report because it works in every way for making that report more powerful. For instance, a report on the Middle East could cite opinions of Henry Kissinger in order to explain its geopolitical significance.
Intelligence analysts verify and re-verify data multiple times to confirm that it is true. For example, a country’s nuclear weapons development report may contain specific data and historic records of their tests with multiple cross-verifications to allow the claimed authenticity and reliability.
These intelligence reports grounded and supported the making of important government and military decisions once completed. A study found that intelligence reports can increase decision accuracy by more than 80% (yes really).
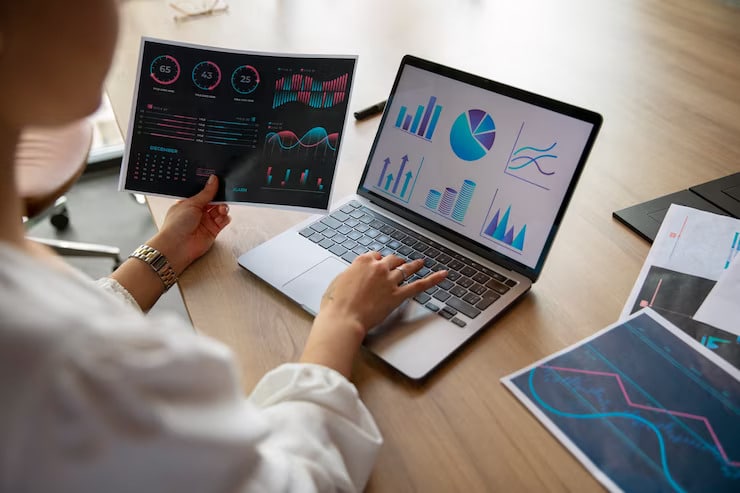
Team Collaboration
A team of 10-15 analysts in intelligence departments who handle thousands of pieces of intelligence every day.
Team members are a part of the operations that analyze strategic and political intelligence with clear roles. One group might relate to the military movements of a country, whilst the other may focus on its economy. This one is from the CIA itself: It reports that team-based analysis can increase accuracy in intelligence interpretation rates by up to 30 percent as per figures.
Its team members meet regularly and exchange the latest information, analysis results (such as what can be shared within public offices in a city) through online collaboration platforms such as Microsoft Teams or Slack. It involves discussion of multiple aspects such as latest geopolitical dynamics, military movements, and economic data within the team members amidst an analysis considering a situation in the Middle East to produce integrative intelligence report.
There is also a way in which team members, when doing intelligence analysis, “herd think” on complex problems—justify desires instead of challenging these conclusions. So, if you, for example, want to analyze how a country is developing its nuclear weapons, team members with different expertise come together and evaluate things in technical ways as well as political or economic. Remember, as former U.S. Secretary of State Henry Kissinger once said: “If you want success in a company, teamwork is the key!”
The reality is the concept of team collaboration matters a great deal. For example, in an intelligence analysis on economic sanctions against a country that required reviewers from five different agencies to approve conclusions line-by-line and some of those analysts were located thousands of miles away close team collaboration enabled analysts to turn around the findings into one week as opposed to two.
They will have interfaces with other countries, as well as the Ministry of Foreign Affairs and the State Department). And they cooperate with a significant number of analytical departments (of which there are at least two), in order to be able to obtain more information resources by joining them. For instance, during one intelligence analysis of international terrorism the department worked simultaneously with counter-terror to identify and access real-time tactical information and resources allowing them to anticipate a potential terrorist attack before it occurred.
CONTACE INFORMATION:
- Aliyun mail: jidong@zhgjaqreport.com
- Blog: https://zhgjaqreport.com
- Gmail:Jidong694643@gmail.com
- Proton mail:Jidong694643@proton.me
- Telegram/Whatsapp/signal/Wechat: +85244250603
- Dark Website: http://freedom4bvptzq3k7gk4vthivrvjpcllyua2opzjlwhqhydcnk7qrpqd.onion