CONTACE INFORMATION:
- Aliyun mail: jidong@zhgjaqreport.com
- Blog: https://zhgjaqreport.com
- Gmail:Jidong694643@gmail.com
- Proton mail:Jidong694643@proton.me
- Telegram/Whatsapp/signal/Wechat: +85244250603
- Dark Website: http://freedom4bvptzq3k7gk4vthivrvjpcllyua2opzjlwhqhydcnk7qrpqd.onion
To analyze information, first, collect a large amount of data and verify the reliability of the sources. Next, use statistical analysis tools to process the data. Finally, combine historical context and real-life cases to conduct a comprehensive evaluation and interpretation.
Understanding Background Information
Because it greatly affects the efficiency and effectiveness of everything else, it becomes very important to understand all other content in the given situation (or at least most of it) in the background. Therefore, for example, if you are investigating political turmoil in a country, you need to understand the history of key political events and economic indicators over the past 10 years.
Take the Cold War as an example. It is essential to understand the stakes of the United States and the Soviet Union in political, military, and economic aspects. Users who do not remember history are destined to inevitably relive it. In the intelligence community, analyzing historical events can prevent mistakes in current decision-making, which is especially true.
Background and Recommendations – Ensure you understand your sources and verify the information collected. For example, in 2019, American economist Joseph Stiglitz pointed out that media reports on U.S. economic growth often overlooked the widening gap between rich and poor.
Intelligence analysis is a professional field rich in terminology. For example, when studying international trade, you need to understand concepts such as “tariff barriers” and “trade deficit.“
In terms of background knowledge, you need to summarize various types from actual cases. For example, when the Ukraine crisis broke out in 2014, analysts were able to learn a lot by studying how the former Yugoslavia collapsed.
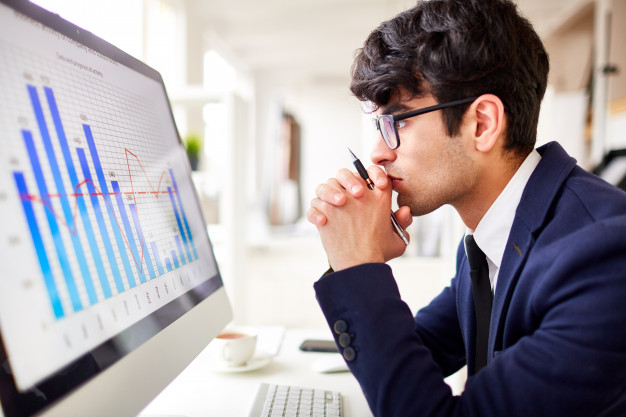
Collecting Data and Information
Good intelligence requires good and extensive data collection. For example, the assessment of China’s economic growth potential: “Last year, China’s GDP exceeded $14.34 trillion, currently accounting for 16.3% of global GDP.”
Industry-specific terminology and concepts must be used to collect data. Note that, for example, in political intelligence analysis, you must be familiar with “election cycles,” “political stability,” and “foreign policy.”
The 2016 Brexit referendum is a typical example. This work involved collecting voting data, opinion polls, and economic forecasts to provide rich information, making it easy to track how Brexit might affect the UK or the EU.
As the famous investor Warren Buffett once said, “Making decisions without data support is gambling.” From the perspective of intelligence analysis, scientific and reasonable judgments cannot be made without collecting all the data.
Common questions should be supported by data or facts. Someone might wonder how to understand a country’s political stability. The answer is to check quantifiable indicators, such as the frequency of political events, government replacement periods, and social turmoil indices.
Data needs to be organized from multiple aspects, such as official statistics, company research reports, media news (including various new media), and various industry analyses. On this topic, government assessments of military strength might include: the country’s own announced annual defense budget; national and foreign defense capability analysis reports published by departments such as the Center for Strategic and International Studies (CSIS) or the International Security Program (ISP); or evaluation reports provided by military experts of specific countries on their websites.
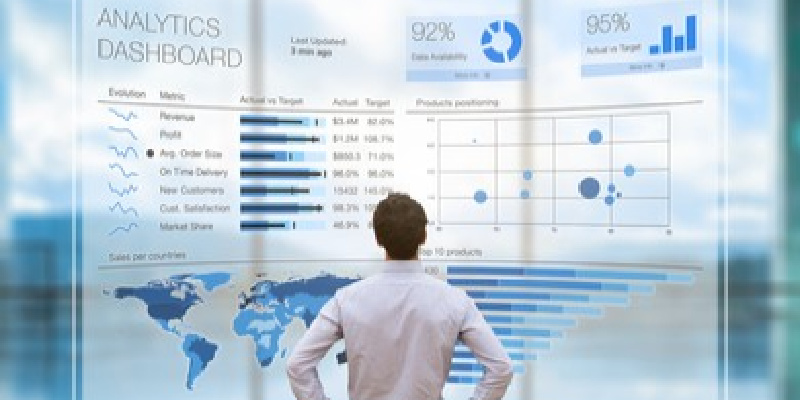
Evaluating and Interpreting Information
This process includes analyzing and interpreting the data, while also assessing whether you believe this is an accurately captured dataset. For example, in 2018, the International Monetary Fund stated that China’s economy grew by about 6.6%, while some unofficial data showed a slightly lower growth rate.
When evaluating data, we must consider the specific concepts and industry terminology used. For example, in terms of political stability, when you want to dissect this idea itself, it is best to separate “politics” and “stability,” such as the frequency of government changes or social protests.
Furthermore, it is crucial to combine real cases and historical events to evaluate and interpret information. The same goes for national economic data, which can change overnight, such as during the 2008 global financial crisis.
Improve the meaning of information by using popular quotes. As Einstein said, “In the midst of difficulty lies opportunity.” Intelligence analysis can be used to predict future changes because it relies on data trends and patterns.
If you encounter specific and accurate queries, it means using a large amount of facts and information to decode. For example, how to measure the health of a country’s economy. The solution lies in examining a set of measurable indicators, such as growth rate, unemployment rate, and inflation level or a country’s foreign exchange reserves.
In addition, information collection must focus on the timeframe/trend of the data. For example, when considering U.S. fiscal policy, the deficit may increase in the short term, but over time, this may lead to economic growth and higher employment rates.
CONTACE INFORMATION:
- Aliyun mail: jidong@zhgjaqreport.com
- Blog: https://zhgjaqreport.com
- Gmail:Jidong694643@gmail.com
- Proton mail:Jidong694643@proton.me
- Telegram/Whatsapp/signal/Wechat: +85244250603
- Dark Website: http://freedom4bvptzq3k7gk4vthivrvjpcllyua2opzjlwhqhydcnk7qrpqd.onion