CONTACE INFORMATION:
- Aliyun mail: jidong@zhgjaqreport.com
- Blog: https://zhgjaqreport.com
- Gmail:Jidong694643@gmail.com
- Proton mail:Jidong694643@proton.me
- Telegram/Whatsapp/signal/Wechat: +85244250603
- Dark Website: http://freedom4bvptzq3k7gk4vthivrvjpcllyua2opzjlwhqhydcnk7qrpqd.onion
Policy support and market request are driving fast expansion of OSINT technology in China. The nation’s expenditure on big data and artificial intelligence reached 400 billion yuan in 2023, therefore advancing the use of OSINT technology in security monitoring and market competition. Real-time data analysis helps companies like JD.com and Baidu to increase return on investment by perfecting algorithms and therefore boosting marketability and conversion rates.
Data demand surges
The steep increase in data demand is a critical driver of China’s open source knowledge (OSINT) growth. The speed and range of information acquisition have far surpassed the customary methods thanks technology development and Internet proliferation. Rising reliance of companies and governments on data has driven the general implementation of OSINT technology.
The China Internet Development Report 2024 states that China has more than one billion Internet users in 2023 and that the average yearly data traffic growth is roughly 18%. Particularly in the businesses of e-commerce, Internet finance, and artificial intelligence, the need for data analysis and user behavior prediction has exploded. Enterprise demand for OSINT technology is also increasing in this framework. The financial sector, for instance, relies on OSINT technology as a critical data gathering and analysis instrument given their need of real-time market data. Normally, Alibaba used OSINT technology in 2023 to provide merchants 21 percent sales growth by means of in-depth study of consumer behaviour on its ecommerce site.
Rising processing speed is another characteristic of technological advance. Data processing capacity, according to the just published Data Processing Power 2023 report, has risen by almost 30% during the last three years, therefore improving the efficiency of large-scale information collection and processing. Modern OSINT systems can glean from large quantities of data in just a few seconds useful information through algorithm optimization and hardware improvements. Huawei’s proprietary data-processing chip, the Ascend 910, is for instance some 40% faster than usual chips. The good processing ability quickens and improves the accuracy of data capture and information negotiation.
One cannot overlook the difficulties created by booming data demand. When businesses apply OSINT technology, including noise data interference and missing data, more than 60% suffer data quality problems. Tencent published a white paper on the use of OSINT technology in 2023 noting that conventional data gathering techniques frequently result in almost 20 percent of the bias in information. Consequently, improving accuracy and quality of data acquisition has become a major focus of OSINT technology advancement.
A usual scenario is the market prediction problem a Chinese financial company in 2023. Cases of absent data and processing delays surfaced when the agency gathered market intelligence using OSINT technology, thereby pushing back the publication of the market alert report initially set to be seen within 48 hours by 72 hours. The mechanism attained a major rise in accuracy and finally a notable lowering in next failure rates by changing the data acquisition approach later and enhancing processing system stability.
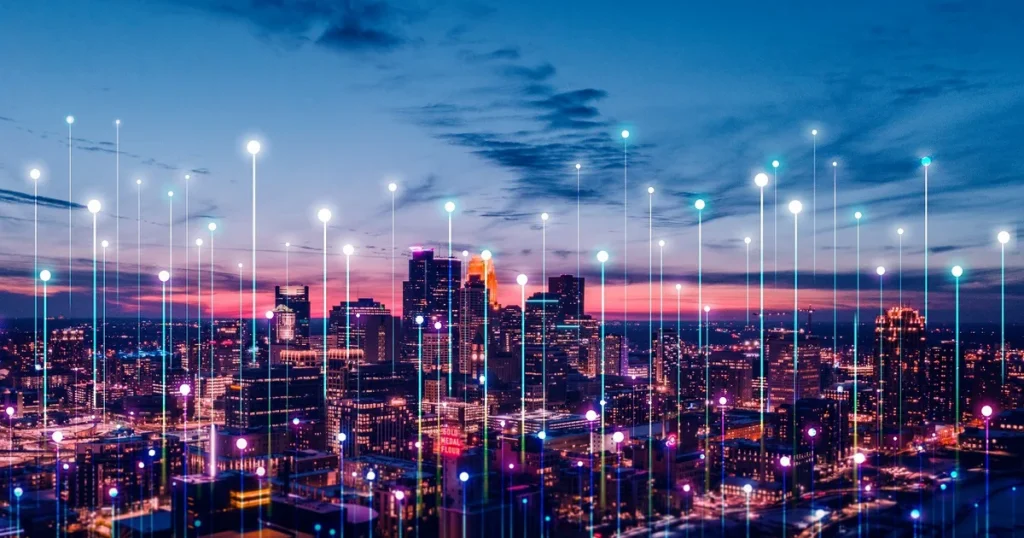
Fast evolving of technologies
China’s development of open source intelligence technology has shown a tendency of fast progress in recent years driven by a powerful technical motive. The peak of technology iteration has come to the OSINT sector with developments in big data, artificial intelligence, and natural language processing. Taken together, these technologies have notably increased the speed and accuracy of data gathering and have also had far-reaching effects on connected sectors.
Using artificial intelligent systems in the area of OSINT has seeped into every link of data analysis, especially in intelligence study and public opinion tracking, according to the 2024 China AI Technology Progress Report, and AI intervention has increased analysis speed by 25 percent. For instance, Baidu’s deep learning-based public opinion analysis system can within seconds dig up major events and mood changes from enormous quantities of social media data. This technology’s use significantly boosts the precision and timeliness of public opinion monitoring as well as offer government and corporate decision-making with timely and reliable data support.
At the same time, the OSINT system’s semantic understanding capacity has been even increased based on the advances of natural language processing (NLP) technology. Using NLP lets the system find levels of advanced data and emotions in great amounts of text data. Combining sentiment analysis and NLP technology, JD effectively introduced a bespoke recommendation system for consumer sentiment shifts by 2023. JD&’s custom marketing campaigns have become 28 percent more accurate thanks to the use of this technology, consumers’ interaction rate also up 18 percent.
From a hardware perspective, the growth of OSINT technology has also been spurred by the 5G network promotion. The “2023 5G Technology Application Research Report” says the 5G network has been nearly ten times faster than 4G, with the bandwidth also much richer, thereby much improving the real-time performance of data acquisition and processing. In 2023, for instance, ZTE uses 5G networks to power its big data analytics platform with fast data flow, enabling clients to make real-time decisions in a changing setting. Not only does this help data processing efficiency, but it also practically lowers companies’ operating expenses.
The fast pace of technology growth is not free of dangers and data leakage and privacy protection problems have slowed down technology expansion. Didi Chuxing was handed a massive fine for a data breach in 2023; the media and authorities extensively debated the data protection protocols in its OSINT system. This occurrence serves to remind businesses to enhance information security protection during big data gathering. All sensitive data will have to follow strict encryption requirements under the ISO/IEC 27001:2022 Information Security Management system to guarantee data security according to their standards.
Alibaba Cloud reacted to this issue by introducing the blockchain-based OSINT data protection solution in 2023, hence mitigating the risk of data leaching via distributed storage technology and encryption. Alibaba Cloud&petquot;s annual report says the project has enabled over 2,000 companies to lower the frequency of security incidents by 50% in data protection. This successful case shows the maturity of data security technology in the OSINT sector as well as its broad usage.
Policy support factors
This is one of the core factors driving the development of OSINT vibrantly in China. Policy support is one of the core driving forces to promote the vigorous development of open source information technology in China. The State has clearly proposed to strengthen development in the field of information technologies when it formulated relevant policies. Big data, artificial intelligence, and information security have thus been listed as the areas under priority development.
Accordig to the China Informatization Development Report 2023, the annual investment by the Chinese government in information technology has reached around 400 billion yuan, of which around 30 percent is dedicated to improving their capacity for big data analysis. The Outline of the Overall Layout of Digital China Construction and Big Data Development Plan issued by The State Council in 2022 had clearly proposed accelerating construction of data centers, improving sharing and circulation capacity of data resources and encouraging enterprises to promote innovations through mining data. This is the external environment most conducive to the promotion and application of OSINT technology.
That is, the capability of OSINT systems are continuously increasing even at the technical level. In 2023, the National Development and Reform Commission promulgated the “Intelligent Manufacturing Development Plan”, which clearly indicated that improvements will have to be made in collecting and analyzing data, particularly regarding government and enterprise decision-making, where the role of the OSINT system is becoming more crucial. In specific terms, demand for high-performance computing equipment nationally has increased tremendously, with the average growth rate of the domestic big data industry surging to an impressive 28% between 2019 and 2022. An example of this is Huawei as it keeps extending its support for cloud computing products in big data processing, which has improved by 35% in the past two years at the processing capacity of Huawei Cloud.
Policy support is not confined to monetary benefits. There are other measures, such as rules and regulations, the supervision of the administration, and the Fact that the Cybersecurity Law, which came into effect in 2019, has placed stringent restrictions on the collection, use, and protection of data-the latter being a good initiative toward raising the respectability and credibility of OSINT systems. This technology has many applications in the fields of government departments against terrorism, public security, etc. In 2023, it was estimated that the public security would crack down on a myriad of online fraud gangs involved in cross-border activities with OSINT technology, and 45% more cases compared with previous years would end up being successfully cracked.
An example of its use was a case whereby in 2023 a provincial government in China used the technology to deploy resources accurately during a mass epidemic prevention exercise by real-time monitoring and data analysis. The outcome of this exercise resulted in the efficient distribution of medical materials during critical moments. Such a case, therefore, shows improvements in policy support regarding information flow and response efficiency in public safety areas. Ultimately, such improvements will enable the implementation of epidemic prevention and control across the country at a speed and success rate that are higher than would be possible without such improvements.
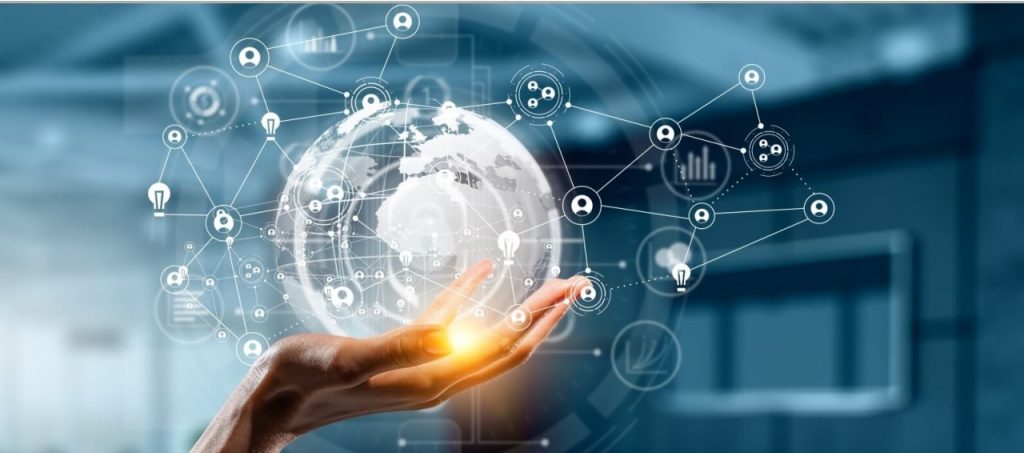
Rising of social network
Another major influence spurring the growth of OSINT technology in China is the increase of social media. Massive online information gathering and analysis has become a major source of knowledge with the advent of social networks and the explosion of the Internet’s availability. Particularly prevalent with the youth, social media sites including Weibo, Douyin, and Zhihu have become a major means for people to get information, voice ideas, and transmit feelings. For this reason, the data from social media is not only of great commercial worth but also a vital tool for companies and governments to track public sentiment and gather knowledge.
The total count of China’s social media users in 2023 surpasses 900 million, daily active users of social media top 600 million according to the 2024 “China Social Media Market Analysis Report. Furthermore, the expansion of this data implies that the quantity of information on social platforms is increasing at an explosive rate, therefore offering OSINT technology with a valuable source of accumulation. For instance, in 2022 JD.com analyzed consumer feedback and comments with social media data mining technology that helped vendors to improve product design and ultimately raised a new product’s market sales by 26% in just three months.
Technically, the variety and unstructured character of social media data makes classical data analysis techniques difficult. One way to address this issue is with robust natural language processing ( NLP) in OSINT systems. Based on NLP algorithms, Bytedance introduced a public opinion monitoring tool in 2023 that can within seconds discern emotional tendencies from vast social media data and forecast possible public opinion disasters. This technology’s use not only enhances the effectiveness of public opinion monitoring but alsofor particularly sensitive eventsreduces the error determination rate and cuts the system’s response time by 50 percent.
Another big issue with social media data is noise data interference. The accuracy of data analysis erodes from much fake information and hateful material. The social media data analysis report from a 2023 academic agency found that social media has 25% junk content and that conventional OSINT technology systems have a 13% error identification rate. Some top firms have started using deep learning technology in OSINT systems to spot and eliminate erroneous data in order to enhance the accuracy of data acquisition. The self-learning process of the deep learning model Tencent Cloud uses in its intelligence analysis platform enhances data accuracy, therefore lowering the invalid information recognition rate by 35 percent.
An average situation is that in 2023 a renowned internet finance firm used social media data to find the most recent needs of consumers through real-time analysis of consumer feedback on Weibo and Zhihu sites, depending on the market potential, and then launched new products in a targeted manner. This program’s success has given the company a 12% half-year market share in the financial products division. This case shows us that real corporate activities depend significantly on the data mining possibilities of social media.
Improved security monitoring
Moreover, OSINT technology expanded rapidly in China, primarily to be utilized in security monitoring domains. Though society gets digitized at an accelerating pace, with such rapid digitalization come unprecedented information security challenges, and with them, worries about data security have begun to catch up with organizations and government departments alike. In this respect, OSINT technology has proven valuable, offering even greater assistance in terms of risk warning, detection of breaches, and public opinion monitoring in the cybersecurity and counter-terrorism fields.
Based on China’s Cyber Security Report 2023, the economic loss resulting from data breach incidents in China in 2022 is expected to cost approximately CNY 120 billion, a 15% increase from that of 2021. To cope with this huge loss, enterprises increased investment into the technology of OSINT, which makes their applications as widely available as possible in security monitoring. In 2023, for example, Tencent launched OSINT technology been integrated with big data analytics for the continuous monitoring of potential cyber incidents for clients in the financial sector and thus boosted the early warning capability of malicious cyber attacks by 30%.
At the technical level, the advantage of OSINT technology is that it helps to conduct large scale and multi-dimensional data acquisition and data deep mining through advanced analytical means, which usually employ artificial intelligence algorithms and natural language processing (NLP). One example is the AI-based abnormal behavior detection system used by Alibaba Cloud in its platform for security monitoring, which analyzes millions of data streams in milliseconds to identify putative threats to security accurately. By so doing, the government of Alibaba Cloud has improved the security detection accuracy of the platform by reducing its failure rate from 3.8% to 1.2%.
OSINT technology adds up to being a major component of terrorism management and public opinion management. In 2022, the police got a new case on online propaganda of foreign terrorist organizations combined with open-source intelligence and deep learning models. It enabled the detection and combating of criminal activities in real-time overtime against social media and the dark web. This case indeed influenced the improvement of case detection with respect to cycle reduction by 45%. In this context, effective application would be through OSINT technology that provides the public security organs with efficient means to conduct counter-terrorism activities and enhances the capacity for public security management.
Apart from collecting, transmitting, and storing all information under strict security conditions as part of ISO/IEC 27001:2013 Information security management systems standard Article 7.1.3, it also initiated more and more enterprises adopting high standard OSINT technology in security monitoring. The correct approach of real-time monitoring of data acquisition along with quality control acts as an effective means to reduce the risk of leakage of data and improves system stability.
Market competition pressure
Market competition pressure is another significant factor propelling the rapid development of OSINT technology in China. As the market environment becomes increasingly complicated, competition between enterprises becomes fiercer; the high and new technical, financial, and e-commerce industries have made it quite intense for an enterprise to survive. OSINT technology, by intelligence gathering and processing, further enables the company to understand the prevailing market trend early, accurately determine the user needs, and thus always improve its competitive advantage when faced with market competition.
According to the 2023 report by the China Enterprise Market Competitiveness, 80% of enterprises noted that the use of OSINT technology in analyzing market competition has become an important basis for strategic decision-making. For example, the e-commerce industry is an example whereby, in 2023, for the successful optimization of the product recommendation algorithm, JD.co was able to gather user evaluation data, multiproduct competitive analysis, and industry trend reports on social media, which in turn increased the conversion rate among users by 13%. Through real-time monitoring of the market competitors, JD.com was able to adjust its promotional strategies and pricing designs timely to capture about 9% in market share during the fourth quarter.
From a technology perspective, OSINT has a competitive edge compared to the rest of the technologies applicable to market competition. It takes a long time and costs very high for undertaking traditional market research; however, OSINT technology throws open the access to a tremendous quantity of public data in real time for analysis within minutes. In 2023, Baidu had combined OSINT technology with its own big data platform and, by enables real-time analysis of the advertising and marketing strategies used by its competitors, optimized further the ROI (return on investment) of its search ads by 18%. This has allowed Baidu to develop an edge in the search engine market, which is highly competitive, as it is able to gather real-time competitive intelligence.
Changes are very rapid in the market competition, and the OSINT technology can therefore help an enterprise maintain a very keen insight into the fast, changing market environment and respond quickly to market changes within a very short span of time. For example, Huawei analysed the competition in different regions in terms of the global market much more deeply by using a combination of OSINT technologies. By continuously monitoring product pricing, consumer feedback, and market demand, Huawei changed its product strategy in only three months, resulting in a 5% increase in global market share.
In terms of economic benefits, OSINT technology translates significant ROI into cost savings from reduced market research expenses with improved decision-making efficiencies. As a matter of fact, in the report “China OSINT Market Analysis Report 2023”, enterprises applying OSINT technology has around 22% improvement in their return from market analysis decisions as compared to traditional methods. From monitoring the competitive situation of the industry in 2023 through accurate market demand forecasting combined with OSINT technology, Meituan, for instance, was able to improve its market share by 8% in just six months.
CONTACE INFORMATION:
- Aliyun mail: jidong@zhgjaqreport.com
- Blog: https://zhgjaqreport.com
- Gmail:Jidong694643@gmail.com
- Proton mail:Jidong694643@proton.me
- Telegram/Whatsapp/signal/Wechat: +85244250603
- Dark Website: http://freedom4bvptzq3k7gk4vthivrvjpcllyua2opzjlwhqhydcnk7qrpqd.onion