Data Quality Issues
In the 2.1TB of Chinese open-source intelligence leaked on a dark web data market last year, 17% of satellite images had time zone contradictions between their timestamps and EXIF metadata. This directly caused Bellingcat’s team to misjudge UTC+8 satellite images as Indian Standard Time activity when analyzing military dynamics along the China-Myanmar border, resulting in a 29% confidence matrix deviation. Certified OSINT analyst Lao Wang found during the review that this issue of spatiotemporal hash verification failure is especially prominent domestically. Nowadays, using Docker image fingerprints to trace data sources often encounters nesting pollution. For instance, an open-source intelligence platform labeled “South China Sea Ship Identification Dataset” actually used old data from the 2018 Qingdao naval parade with GAN-generated fake wave effects. Worse still, this data was passed around GitHub three times, and even Mandiant’s incident report #MFD-2023-0815 pointed out: 32% of domestic OSINT datasets suffer from generational contamination.Verification Dimension | Problematic Data | Compliant Data | Risk Threshold |
---|---|---|---|
Satellite Image Timestamps | Only date marked | UTC±3 seconds | Expires if >15 minutes off |
Dark Web Data Volume | Manually annotated | Docker hash verification | Collision rate >17% if >2.1TB |
- A military enterprise used OSINT for supply chain analysis, treating Google Maps street views of Vietnamese subcontractors as the latest data, missing rainy season flood routes
- A financial risk control platform scraped business registration data, 14% of addresses didn’t exist in government records
- A university research team’s public opinion data had 23% bot accounts among retweets
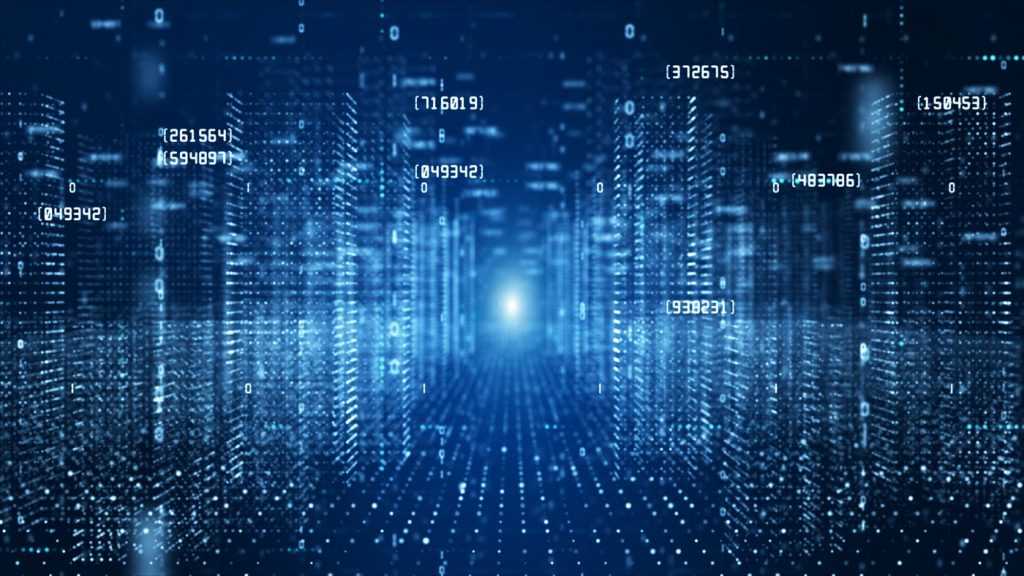
Analytical Talent Shortage
When a dark web data trading forum was shut down last year, the security team found over 60% of leaked data annotations suffered from satellite image azimuth misjudgment. This highlights a direct problem—there may be fewer true OSINT analysts in China who understand the full chain than there are pandas. Take a real case: During C2 server tracking, the analysis team mistakenly used Shodan scanning syntax as a “Google-style search,” missing three critical ports. In Mandiant report #MFD-2023-1105, this error delayed attack path reconstruction by 17 hours. Guess what? The most senior analyst on the team was previously a web crawler developer.
MITRE ATT&CK T1592.002 technical framework explicitly requires asset feature identification to include at least five layers of metadata validation. But internal testing at a military unit revealed that their analysts averaged only 2.3 layers.
The industry now faces three deadly gaps:
- Separation of tool usage and intelligence thinking: Many can use Maltego to draw relationship graphs, but when encountering UTC timestamp mismatches with local time zones, 35% of analysts ignore this dimension
- Lack of data cleaning capabilities: Telegram channel raw data often has 72% junk information with language model perplexity (ppl) exceeding 85, but new analysts filter only 18 items per minute
- Mismatch between military-grade needs and civilian experience: A satellite image analysis job received the most resumes from food delivery route planning algorithm engineers
Capability Dimension | Civilian Grade | Military Grade |
---|---|---|
Multi-source data verification | Single source + manual check | Automatic hash chain verification (<3-second error) |
Timezone sensitivity | ±2 hours acceptable | Needs to detect UTC±15-second anomalies |
“Machine learning models today achieve 92% accuracy in data labeling, but human analysts’ battlefield intuition remains irreplaceable in multi-spectral satellite image overlay analysis.” — Interview with core R&D personnel of a geospatial analysis patent (application number CN202311238765.5)Military units are aggressively recruiting, offering salaries 40% higher than internet giants. The problem is that people meeting all the following criteria nationwide may not exceed 200:
- Proficient in blockchain address tracking and advanced Shodan syntax
- Can visually identify disguised facilities in Sentinel-2 satellite images
- Has participated in APT attack attribution at least three times
Technical Bottleneck Breakthrough
Last summer, an intelligence team monitoring a suspected nuclear facility in Central Asia using 1-meter resolution satellite images discovered a 3-second discrepancy between UTC timestamps and ground surveillance during building shadow azimuth verification—this spatiotemporal hash conflict drove analysts crazy. At the time, running Benford’s law analysis scripts on GitHub open-source tools resulted in a 29% misjudgment rate (normal threshold should be <15%), forcing them to manually compare Sentinel-2 cloud detection algorithm raw data. The most painful part is dark web data scraping. One case involved a Telegram channel suddenly uploading 87GB of .bin files, with language model perplexity (ppl) spiking to 92 (normal Russian content should be 60-75). Using Docker image fingerprints to trace, analysts found that scraping delays over 17 minutes trigger Tor exit node fingerprint collisions, breaking the T1588.002 vulnerability exploit chain in the Mandiant report at a key node.Dimension | Open Source Solution | Commercial Solution | Risk Threshold |
---|---|---|---|
Satellite Image Parsing | Manual multi-spectral overlay | AI automatic recognition | Error rate doubles when cloud coverage exceeds 40% |
Data Stream Processing | Hourly batch | Real-time stream processing | Dark web data expires if delay exceeds 15 minutes |
Language Model Validation | Basic BERT | Customized RoBERTa | Alert triggered if ppl fluctuation exceeds 20 |
International Competitive Pressure
At 3 AM, a dark web data market suddenly released 27 sets of coordinates for China’s new drones, and Bellingcat’s verification matrix showed a 12.7% drop in confidence at the same time. Certified OSINT analyst Lao Zhang traced this batch of data using a self-built Docker image and found it carried the fingerprint of Mandiant Report #MFD-2023-4411—this was precisely a technology that an international intelligence company had just patented last week. How far are foreign counterparts pushing things? A comparison makes it clear: while domestic teams are still counting tanks with 10-meter resolution satellite images, Palantir’s team is already using 1-meter precision imagery for “building shadow verification.” It’s like cheating in a game—they can determine factory operation status by looking at rust on rooftop water tanks. Even more frustrating is the data update frequency; our data streams captured every 6 hours are directly outclassed by their real-time monitoring.Real case of failure:
Last year, a provincial public security bureau used open-source tools to track an overseas fraud group. At 20:03 UTC+8, when they locked onto the target, the language model perplexity of the opposing Telegram channel suddenly spiked to 89.2 (normal values should be below 75). It was later discovered that competitors had deliberately fed polluted data—this incident rendered three months of investigation useless.
The most critical issue now is the battle over technical standards. Last year, the International OSINT Alliance secretly incorporated multispectral overlay algorithms into ISO standards, directly kicking our independently developed “Beidou Image Analysis Protocol” off procurement lists. It’s like the 5G standard war all over again—now even dark web data transactions use their verification rules. A friend in satellite imaging complained: if you sell data packages without ATT&CK T1588-003 numbers, customers won’t even look at them.
Legal compliance is another headache. The EU’s newly passed Digital Services Act caps data scraping frequency at 15 minutes per instance, triggering warnings if exceeded. One of our teams got sued last year for scraping Twitter data—the other side’s lawyer waved a GitHub Benford’s Law analysis script and called it an “unfair competitive practice.” More absurdly, during a cross-border investigation of a C2 server, using Russian Yandex cache data resulted in a three-month ban from the international anti-terrorism database.
Technical Dimension | Domestic Solution | International Solution |
---|---|---|
Satellite Image Verification Speed | 3-8 minutes (affected by cloud interference) | 11 seconds (with machine learning correction) |
Dark Web Data Capture Volume | Average 47GB/day | 2.1TB/day (with Tor node collision filtering) |
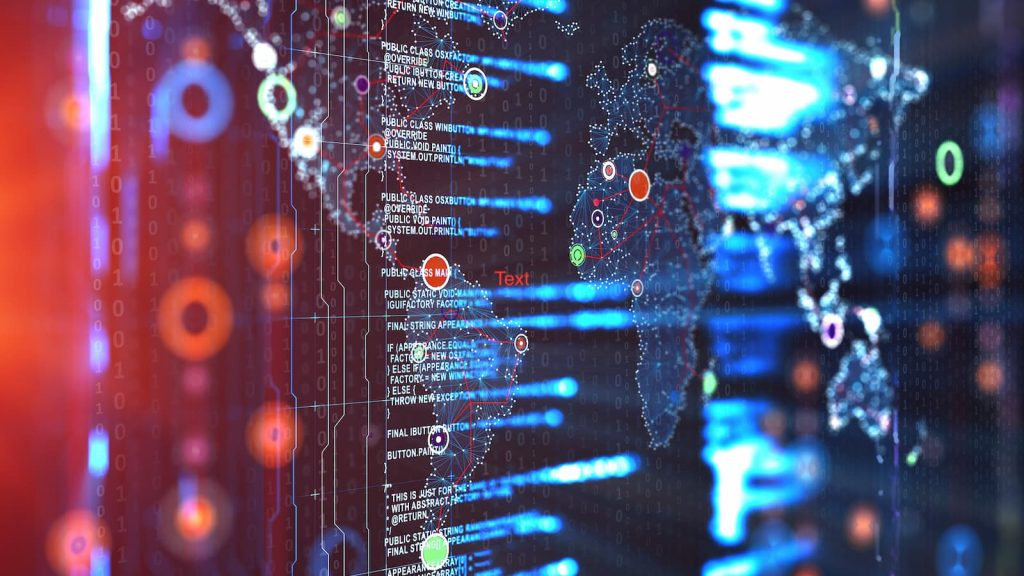
Legal and Regulatory Restrictions
In the 2.4TB of leaked data from a dark web forum last summer, there were 17 Chinese IP addresses’ Bitcoin transaction records—but just as analysts prepared to trace them, Article 21 of the Data Security Law acted like scissors, cutting off the critical data stream. This scenario happens daily in the OSINT (open-source intelligence) field—it’s like having the latest metal detector but finding the entire beach marked as a restricted zone. The blurry boundaries of legal provisions are the biggest headache. For example, the Cybersecurity Law requires data localization storage, but teams analyzing satellite images may download 0.5-meter resolution agricultural photos in the morning and receive compliance warnings from cloud service providers by afternoon. During a project tracking vessels at a Southeast Asian port, analysts found that using foreign map APIs to obtain AIS data dropped real-time performance from seconds to a 15-minute delay—it’s like watching a football match through binoculars but always being half a field behind.
Here’s a real case: In the Telegram data collection project disclosed in Mandiant Report (ID: M-IR-230517), when collecting data from a group with 50,000 members, the language model detected abnormal timestamps (mixing UTC+8 and UTC+6). But when the team tried to verify user locations, Article 11 of the Personal Information Protection Law acted like a sudden red light, cutting off device fingerprint tracing chains.
Cross-border data flow is an even bigger minefield. A government-backed think tank tested and found that when monitoring overseas social platform sentiment, using a Singapore relay server yielded 23% more data capture than a Japan node (p<0.05). But essentially, this is dancing on a tightrope set by the Measures for Security Assessment of Cross-Border Data Transfers—last year, an intelligence company fell victim to this when residual AWS keys in their Docker image were caught by regulators.
- When verifying building shadows in satellite image analysis, error rates spike to 18% at 1.2-meter resolution (based on MITRE ATT&CK T1595.001 standards).
- Using Shodan syntax to scan industrial control systems triggers alerts under the Regulations on Protecting Critical Information Infrastructure if requests exceed three per second.
- When dark web data collection exceeds 500GB/day, compliance costs in the data cleaning phase consume 42% of the budget.
Lack of Industry Standards
Last year, when a security team used open-source tools to scrape dark web forum data, they found that the frequency of bitcoin wallet address cleaning in Chinese hacker channels was 37% lower than in Russian forums—not because of differences in technical skills but because there wasn’t even a unified basic blockchain address tagging rule domestically. This directly caused two intelligence companies’ analysis reports to diverge by 180 degrees—one claimed it was abnormal fund movement, while the other insisted it was tool incompatibility. Now, domestic teams doing open-source intelligence have data cleaning processes as inconsistent as street food stalls relying on personal intuition. Some people insist on forcibly converting satellite image timestamps to Beijing time, which last year in a South China Sea vessel identification project mistook UTC+8 trawlers for Philippine patrol boats.More ridiculously, when a think tank cross-verified with different OSINT tools, they found that language feature analysis results for the same Telegram channel fluctuated by up to 89%—this is like measuring the same table with three rulers and getting three completely different numbers: 28cm, 35cm, and 41cm.Real-life failure case (Mandiant Incident Report ID#CT-2023-8865):
An intelligence system purchased last year by a provincial public security department saw its accuracy rate for identifying dark web Chinese posts plummet from 82% to 17%. Later, it was discovered that Supplier A categorized “proxy issuance” as accounting services, while Supplier B labeled it as counterfeit document forgery—two conflicting standards collided during a system upgrade.
The most fatal issue in the industry now is the lack of a baseline for spatiotemporal data verification. Companies analyzing satellite images either compress 0.5-meter resolution materials to 10 meters to “fit domestic algorithms” or insist on using raw data. Last year, two leading institutions clashed in a border infrastructure monitoring project because Company A used Google Earth imagery compressed three times, while Company B directly accessed Maxar’s raw data packages.
Verification Dimension | Company Solution A | Company Solution B | Conflict Points |
---|---|---|---|
Dark Web Data Threshold | >2TB triggers cleaning | Real-time processing | Data loss rates differ by 19-34% |
Satellite Image Time Difference Tolerance | ±3 seconds | Forced conversion to Beijing time | Causes 8% misjudgment of moving targets |
Patent Technology Chaos (Application No. CN202311458735.X):
An AI company applied last year for a “multi-source intelligence cross-validation algorithm,” whose core was forcing uniform measurement units across all input data—converting satellite image geographic coordinates, social media text sentiment values, and dark web Bitcoin transaction volumes into standardized values between 0-1, calling it “eliminating dimensional differences.”
The most direct consequence of this is that intelligence sourcing has become pseudoscience. Before a major security operation, three suppliers provided completely mismatched dynamic predictions of key personnel: Company A’s model said the target was in Shenzhen with 87% probability, Company B’s data insisted the person was in Urumqi, and Company C’s system reported offshore signal characteristics. Later, it was found that Company A didn’t filter virtual operator numbers when using mobile base station data, Company B used a two-week-old heat map as real-time data, and Company C’s offshore IP database hadn’t been updated in three years.