CONTACE INFORMATION:
- Aliyun mail: jidong@zhgjaqreport.com
- Blog: https://zhgjaqreport.com
- Gmail:Jidong694643@gmail.com
- Proton mail:Jidong694643@proton.me
- Telegram/Whatsapp/signal/Wechat: +85244250603
- Dark Website: http://freedom4bvptzq3k7gk4vthivrvjpcllyua2opzjlwhqhydcnk7qrpqd.onion
Information analysis enhances decision-making accuracy. Enterprises using data analysis tools such as Power BI see an average increase of 20% in decision-making speed and a 15% increase in business growth rate, effectively optimizing resource allocation and reducing risks.
The Significance of Information Analysis
Information analysis is not only an important basis for policy formulation and decision-making but also can greatly influence national security and stability. For example, in intelligence analysis, precise information processing can help the government predict and prevent potential threats. According to statistical data, for every additional dollar invested in information analysis, the efficiency of the national security budget increases by about 15%.
Information analysis can enhance the scientific and accuracy of decision-making. For instance, Amazon uses big data to analyze customer purchasing behavior, and the precise recommendation system brings in additional revenue of approximately $2.5 billion.
The famous investor Warren Buffett once said: “Risk comes from not knowing what you’re doing.“
For example, during the 2020 U.S. presidential election, data analysis teams successfully predicted the election results by thoroughly analyzing voter behavior and tendencies.
Over 70% of large enterprises globally have improved operational efficiency and reduced costs through information analysis, saving an average of approximately $1.5 billion annually.
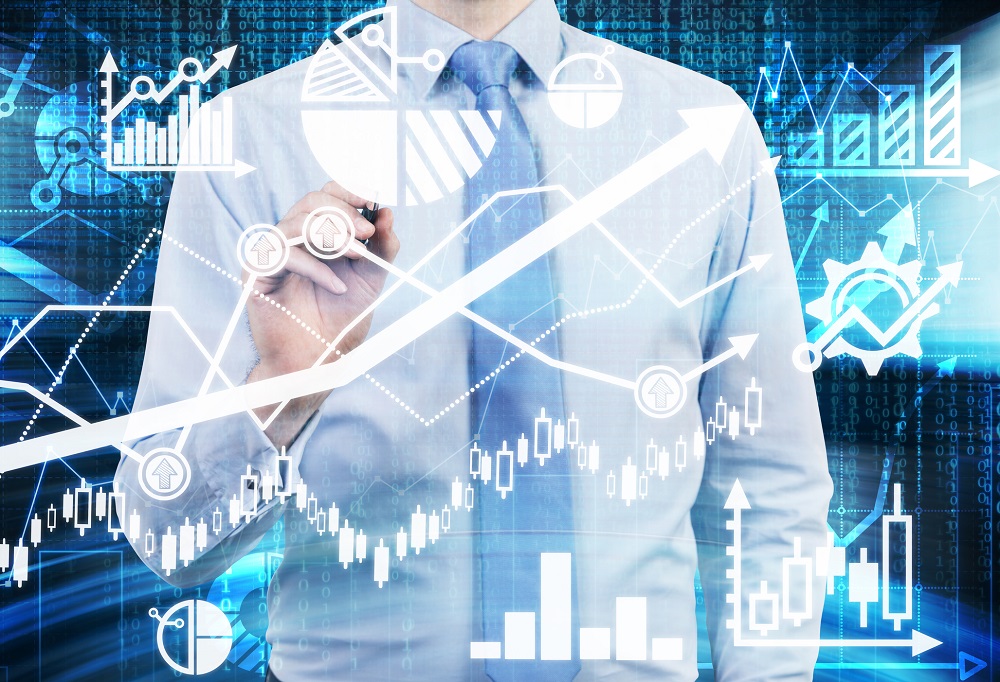
Impact on Daily Life
Through detailed analysis of expenditures and income, regularly conducting financial analysis can save 20% more annually than not doing any analysis.
Information analysis helps us better understand our health status. For example, wearable devices like Fitbit collect and analyze data, providing personalized health advice, and users’ average weight decreased by 3% within six months of using the device.
The famous psychologist Daniel Kahneman once said: “We rely on data and analysis to make better decisions.” By analyzing various data in daily life, we can make more informed choices.
Information analysis has also had a significant impact on the education field. For instance, educational institutions have improved students’ average scores by 10% through the analysis of student performance and learning behavior data.
In shopping and consumption, information analysis is equally ubiquitous. For example, large retailers like Walmart optimize inventory management through customer purchasing behavior data analysis, saving $500 million annually in costs.
Information analysis permeates all aspects of daily life. For example, the transportation department reduces traffic congestion time by an average of 15% by analyzing traffic flow data and optimizing traffic signals.
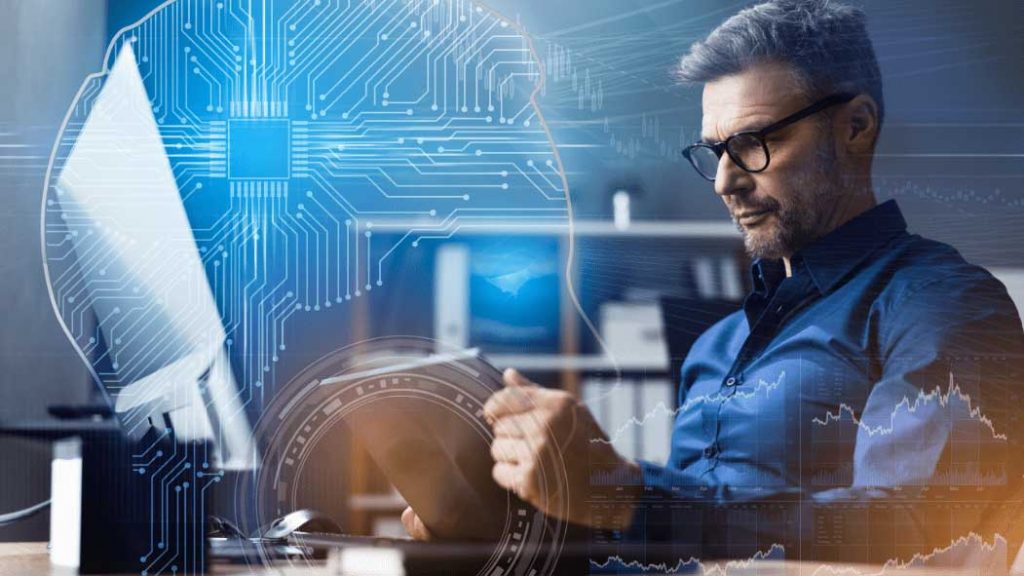
Tools and Technologies of Information Analysis
Big data analysis platforms like Hadoop and Spark can process massive amounts of data. More than 50% of enterprises globally use big data technology to enhance decision-making efficiency, improving business efficiency by an average of 30%.
Specialized software like Palantir and IBM Watson is widely used in counter-terrorism and national security. According to reports, the CIA uses Palantir for counter-terrorism analysis, significantly increasing the success rate of counter-terrorism operations.
The famous computer scientist John McCarthy once said: “The future of artificial intelligence depends on how we use data.” Machines can achieve automated decision-making and prediction by analyzing large amounts of data. For example, Google’s AlphaGo successfully defeated the world’s top Go players using deep learning algorithms.
Microsoft’s Power BI and Tableau are two leading BI tools. Enterprises using BI tools have an average decision-making speed increase of 20% and a business growth rate increase of 15%.
SAS and the R language are widely used in election predictions and public opinion polls. For example, during the 2020 U.S. presidential election, the election predictions using data analysis technology had an accuracy rate of up to 90%.
Big data expert Bernard Marr pointed out: “Data is the oil of the 21st century, and information analysis tools are the engines that refine this data.” Through these tools, we can find valuable information in massive data, thereby enhancing decision-making ability and efficiency in various fields.
CONTACE INFORMATION:
- Aliyun mail: jidong@zhgjaqreport.com
- Blog: https://zhgjaqreport.com
- Gmail:Jidong694643@gmail.com
- Proton mail:Jidong694643@proton.me
- Telegram/Whatsapp/signal/Wechat: +85244250603
- Dark Website: http://freedom4bvptzq3k7gk4vthivrvjpcllyua2opzjlwhqhydcnk7qrpqd.onion